Open Access
ARTICLE
Coot Optimization with Deep Learning-Based False Data Injection Attack Recognition
1 Department of Information Technology, Chaitanya Bharathi Institute of Technology, Hyderabad, Telangana, India
2 Department of Computer Science and Engineering, Aditya Engineering College, Surampalem, Andhra Pradesh, India
3 Department of Electrical Engineering, College of Engineering, Jouf University, Saudi Arabia
4 Department of Computer Science and Engineering, Vignan’s Institute of Information Technology, Visakhapatnam, 530049, India
5 School of Electrical and Electronic Engineering, Universiti Sains Malaysia, Nibong Tebal, 14300, Malaysia
* Corresponding Author: Mohamad Khairi Ishak. Email:
Computer Systems Science and Engineering 2023, 46(1), 255-271. https://doi.org/10.32604/csse.2023.034193
Received 08 July 2022; Accepted 11 October 2022; Issue published 20 January 2023
Abstract
The recent developments in smart cities pose major security issues for the Internet of Things (IoT) devices. These security issues directly result from inappropriate security management protocols and their implementation by IoT gadget developers. Cyber-attackers take advantage of such gadgets’ vulnerabilities through various attacks such as injection and Distributed Denial of Service (DDoS) attacks. In this background, Intrusion Detection (ID) is the only way to identify the attacks and mitigate their damage. The recent advancements in Machine Learning (ML) and Deep Learning (DL) models are useful in effectively classifying cyber-attacks. The current research paper introduces a new Coot Optimization Algorithm with a Deep Learning-based False Data Injection Attack Recognition (COADL-FDIAR) model for the IoT environment. The presented COADL-FDIAR technique aims to identify false data injection attacks in the IoT environment. To accomplish this, the COADL-FDIAR model initially pre-processes the input data and selects the features with the help of the Chi-square test. To detect and classify false data injection attacks, the Stacked Long Short-Term Memory (SLSTM) model is exploited in this study. Finally, the COA algorithm effectively adjusts the SLTSM model’s hyperparameters effectively and accomplishes a superior recognition efficiency. The proposed COADL-FDIAR model was experimentally validated using a standard dataset, and the outcomes were scrutinized under distinct aspects. The comparative analysis results assured the superior performance of the proposed COADL-FDIAR model over other recent approaches with a maximum accuracy of 98.84%.Keywords
Cite This Article
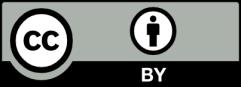
This work is licensed under a Creative Commons Attribution 4.0 International License , which permits unrestricted use, distribution, and reproduction in any medium, provided the original work is properly cited.