Open Access
ARTICLE
SNELM: SqueezeNet-Guided ELM for COVID-19 Recognition
1 School of Computing and Mathematical Sciences, University of Leicester, Leicester, LE1 7RH, UK
2 Department of Computer Science, HITEC University Taxila, Taxila, Pakistan
* Corresponding Author: Shuihua Wang. Email:
Computer Systems Science and Engineering 2023, 46(1), 13-26. https://doi.org/10.32604/csse.2023.034172
Received 07 July 2022; Accepted 22 September 2022; Issue published 20 January 2023
Abstract
(Aim) The COVID-19 has caused 6.26 million deaths and 522.06 million confirmed cases till 17/May/2022. Chest computed tomography is a precise way to help clinicians diagnose COVID-19 patients. (Method) Two datasets are chosen for this study. The multiple-way data augmentation, including speckle noise, random translation, scaling, salt-and-pepper noise, vertical shear, Gamma correction, rotation, Gaussian noise, and horizontal shear, is harnessed to increase the size of the training set. Then, the SqueezeNet (SN) with complex bypass is used to generate SN features. Finally, the extreme learning machine (ELM) is used to serve as the classifier due to its simplicity of usage, quick learning speed, and great generalization performances. The number of hidden neurons in ELM is set to 2000. Ten runs of 10-fold cross-validation are implemented to generate impartial results. (Result) For the 296-image dataset, our SNELM model attains a sensitivity of 96.35 ± 1.50%, a specificity of 96.08 ± 1.05%, a precision of 96.10 ± 1.00%, and an accuracy of 96.22 ± 0.94%. For the 640-image dataset, the SNELM attains a sensitivity of 96.00 ± 1.25%, a specificity of 96.28 ± 1.16%, a precision of 96.28 ± 1.13%, and an accuracy of 96.14 ± 0.96%. (Conclusion) The proposed SNELM model is successful in diagnosing COVID-19. The performances of our model are higher than seven state-of-the-art COVID-19 recognition models.Keywords
Cite This Article
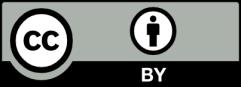
This work is licensed under a Creative Commons Attribution 4.0 International License , which permits unrestricted use, distribution, and reproduction in any medium, provided the original work is properly cited.