Open Access
ARTICLE
Behavioral Intention to Continue Using a Library Mobile App
1 School of Management, Wuhan Institute of Technology, Wuhan, 430205, China
2 Department of Business Administration and Management, Hungarian University of Agriculture and Life Sciences, Godollo, 2100, Hungary
* Corresponding Author: J. R. Ming. Email:
Computer Systems Science and Engineering 2023, 46(1), 357-369. https://doi.org/10.32604/csse.2023.033251
Received 12 June 2022; Accepted 30 September 2022; Issue published 20 January 2023
Abstract
To meet the needs of today’s library users, institutions are developing library mobile apps (LMAs), as their libraries are increasingly intelligent and rely on deep learning. This paper explores the influencing factors and differences in the perception of LMAs at different time points after a user has downloaded an LMA. A research model was constructed based on the technology acceptance model. A questionnaire was designed and distributed twice to LMA users with an interval of three months to collect dynamic data. The analysis was based on structural equation modeling. The empirical results show that the perceived ease of use, the perceived usefulness, the social influence, and the facilitating conditions affected the users’ behavioral intention, but their impacts were different at different times. As the usage time increases, the technology acceptance model is still universal for understanding the user perception of LMA. In addition, two extended variables (social impact and convenience) also affect the user’s behavior intention. User behavior is dynamic and changed over time. This study is important both theoretically and practically, as the results could be used to improve the service quality of LMAs and reduce the loss rate of users. Its findings may help the designers and developers of LMAs to optimize them from the perspective of a user and improve the service experience by providing a deeper understanding of the adoption behavior of information systems by LMA users.Keywords
With modern information technology and mobile networks developing rapidly, people are now accessing information from many different types of mobile device. The trend for libraries is that they will change from the traditional service mode of library borrowing to that of offering reader-centered intelligent knowledge based on artificial intelligence. For example, intelligent recommendation systems are being developed. Many colleges and universities consider that library mobile apps (LMAs) cannot be ignored. Such apps offer new library services that extend the traditional library services, allowing users to obtain information and services through mobile devices anywhere at any time. An LMA fully incorporates the basic functions of a library by exploiting the features of mobile communications to provide intelligent services based on location that are context aware. As new platforms for sharing knowledge and learning, LMAs offer information about library catalogs, databases, open classes, and library guides, among others. They are a new service model that will improve the efficiency of the users’ daily life and learning. Zhang [1] found that 92% of 39 well-known universities in China have constructed LMAs, and Xu et al. [2] found that 27 out of 42 universities in China offering major subjects have built LMAs.
In China, LMAs have developed quickly and smoothly, and many people have downloaded and tried them. However, the acceptance and adoption of LMAs is relatively low [3], and relatively few university students continue to use them for accessing library services. In other words, users’ stickiness to LMAs is not high. Consequently, the development of LMAs has not achieved the expected results. Jasperson et al. [4] believe that the success of an information system depends on whether users continue to use it. The initial adoption of an information system is only the first step. If users do not continue to use it, it will not bring them the expected usage value. Similarly, whether someone continues to use an LMA after initial adoption determines the success or failure of the LMA. Therefore, it is necessary to conduct dynamic-tracking research on the user perception behavior (UPB) of LMAs.
LMAs have attracted extensive research attention, mainly focusing on their basic theory [5,6], their technology and service models [7,8], their development status [9,10], or user behavior [11–14]. However, the success of an LMA depends on its continued use, so it is necessary to explore the behavior of user after they initially adopt an LMA. Dynamic-tracking research with Chinese users will offer deeper insights into UPB and provide important considerations for the developers and designers of LMA services.
The present paper is focused on the UPB of an LMA. Based on the technology acceptance model (TAM), we tracked LMA users over time. At two different time points (T1 and T2), three months apart, we asked the LMA users to complete a structured questionnaire. We then explored the influencing factors and dynamic changes in the UPB of the LMA in these real situations. This study aimed to obtain an accurate picture of user behavior when interacting with an LMA, which could be used to improve the services offered by an LMA. The study addressed the following two main research questions (RQs):
RQ1. What is the suitability of TAM for understanding the UPB of LMAs in dynamic research?
RQ2. What are the key factors that affect the UPB of LMAs, and do these factors change over time?
With the rapid development of LMAs, the literature review on the user adoption and acceptance of LMAs was helpful in clarifying research results for the UPB of LMAs.
Researchers have explored the factors that influence the user adoption of LMAs. Jaradat [15] constructed a research model based on TAM and incorporated other variables. The results showed that perceived usefulness (PU) and perceived ease of use (PEOU) were the main factors affecting user acceptance and usage of LMAs. Aharony [16] built a research model based on TAM and introduced individual innovation and media variables. The results showed that PEOU and individual innovation affected user adoption of LMAs. By using the unified theory of acceptance and use of technology (UTAUT), Saravani et al. [17] found that, among library managers and librarians in New Zealand and Australia, performance expectations, effort expectations, social influence (SI), and facilitating conditions (FCs) affected users’ behavioral intention (BI) to use LMAs.
Empirical research has also been conducted on users’ BI to use LMAs. Huang et al. [11] integrated TAM and the theory of planned behavior to explore user adoption behavior. Their results showed that PU, PEOU, interpersonal influence, FCs, attitude, subjective norms, and perceived behavioral control were the main factors influencing whether college students used LMAs. Wang et al. [18] constructed a research model based on the theory of diffusion of innovations and DeLone and McLean’s information system success model. They incorporated PU and PEOU into the model and found that these two factors positively affected users’ willingness to use LMAs. Vongjaturapat et al. [19] constructed a user-acceptance research model of LMAs based on UTAUT and the task–technology fit. They incorporated screen design and interactive interface. The results showed that SI, performance expectations, task–technology matching degree, and effort expectations affected users’ actual use of LMAs. Using category theory and TAM, Zhang et al. [20] analyzed the main factors influencing university students’ acceptance of LMAs, and the results showed that SI positively affected users’ willingness to use LMAs.
As can be seen, several researchers have constructed models for user adoption and acceptance of LMAs based on existing theories. The main theories are the theory of planned behavior, TAM, task–technology fit, diffusion of innovations, DeLone and McLean’s information system success model, and UTAUT, of which TAM is used most frequently, followed by UTAUT. In most TAM studies, a cross-sectional approach is used to statically explore the relations between variables. The data are collected from objects for a single time period, so the empirical results can explain the UPB only in that period. However, assessing user perception and feedback about LMAs at a specific time cannot fully reflect the overall use status, as a user’s perception of an LMA can change after a period of use. Neglecting the dynamics of user behavior inevitably reduces the comprehensiveness of the research conclusions [21]. Therefore, researching the UPB of LMAs should be a long-term dynamic process. TAM can be applied to the UPB of LMAs, but whether it remains suitable in dynamic situations is still to be verified.
3 Construction of Research Model
3.1 Technology Acceptance Model
Davis [22] proposed TAM based on the theory of reasoned action and reasoned that PU and PEOU were key variables that affected user acceptance and their use of information systems. That study showed that TAM can effectively explain and predict users’ attitudes toward and their behavior regarding information systems. Because it has a solid theoretical basis, TAM is an effectively universal research model for investigating users’ BI to use an information system. Therefore, we took TAM as the basic theory and incorporated other variables to better explain and predict users’ BI to use an LMA in a new environment.
A student’s perception of an LMA is affected directly by timely publicity, information from colleges and libraries, and usage and promotion by college teachers and other students. In other words, SI significantly affects someone’s willingness to use an LMA. Moreover, in the initial stages of promoting any new technology or service, the ease of use affects user perception and whether they use the new technology. Using an LMA requires access to a mobile device connected to a mobile network with a stable network speed. That is, FCs affect an individual’s willingness to use an LMA. With increasing use time, the UPB of an LMA will vary.
Theoretically, the ideal evaluation method is to use the actual use behavior as the objective measure of the acceptance of information technology or an information system. However, it is difficult to directly observe user behavior in a conventional way. Liaw et al. [23] and Huang et al. [24] found a strong positive correlation between users’ BI and their actual use behavior. Therefore, for measurement convenience, in the present study we used BI instead of actual use behavior. We constructed a UPB model for LMAs based on TAM and incorporated the variables SI and FCs, as shown in Fig. 1.
Figure 1: Research model
In TAM, PEOU is the degree to which a person believes that using a particular system will require little effort. PU is defined as the degree to which a person believes that using a particular system will enhance their performance at some task [22]. Users will tend to use an LMA if they perceive that its interface is simple and easy to operate. However, as use time increases, users will not continue to use an app merely because it is easy to operate if it has limited utility. If people perceive that an LMA is useful to them, such as by significantly enhancing their learning and efficiency, then they will tend to use it. After users acquire more experience, the impact of PEOU on users’ BI to use LMAs will weaken, and the impact of PU will increase users’ BI to use LMAs. PEOU will improve a user’s experience, thus enhancing PU. However, as the use time increases, the impact of PEOU on PU will weaken. Thus, we propose the following hypotheses:
H1a. PEOU has a positive influence on users’ BI to use an LMA.
H1b. Compared with time point T1, the impact of PEOU on users’ BI to use an LMA will be weaker at time point T2.
H2a. PU has a positive influence on users’ BI to use an LMA.
H2b. Compared with T1, the impact of PU on users’ BI to use an LMA will be stronger at T2.
H3a. PEOU has a positive influence on users’ PU of an LMA.
H3b. Compared with T1, the impact of PEOU on the PU of an LMA will be weaker at T2.
For a new system, SI is defined as the degree to which an individual perceives that important others believe that they should use it [25]. If a particular college and its library promote an LMA and relevant teachers, classmates, and friends use and recommend the services provided, then the perception of the usefulness of the LMA is enhanced. Thus, SI has a positive influence on PU. In turn, PU will increase a users’ BI to use the LMA. However, as use time increases, users gradually become more rational, and whether they continue to use the LMA will depend mainly on PU, so that the impact of SI on PU and BI will weaken. Consequently, we propose the following hypotheses:
H4a. SI has a positive influence on users’ BI to use an LMA.
H4b. Compared with T1, the impact of SI on users’ BI to use an LMA will be weaker at T2.
H5a. SI has a positive influence on the PU of an LMA.
H5b. Compared with T1, the impact of SI on the PU of an LMA will be weaker at T2.
FCs are defined as the degree to which an individual believes that an organizational and technical infrastructure exists to support use of the system [25]. Users tend to use an LMA if they have a mobile device (e.g., a smartphone or tablet) and can easily access its services via a stable and cheap mobile network. However, as use time increases, the impact of FCs on the use of the LMA will become more apparent, so the effect will increase. Good FCs will affect PEOU and increasing use time will enhance this relation. Consequently, we propose the following hypotheses:
H6a. FCs have a positive influence on users’ BI to use an LMA.
H6b. Compared with T1, the impact of FCs on users’ BI to use an LMA will be stronger at T2.
H7a. FCs have a positive influence on the PEOU of an LMA.
H7b. Compared with T1, the impact of FCs on the PEOU will be stronger at T2.
5.1 Selection and Characteristics of Respondents
For the present empirical research, we selected university students as the respondents for the following three reasons:
1. Many university students are willing to accept and use LMAs, and they are the main users of LMAs.
2. The present research on user adoption of an LMA relies on structured questionnaires to obtain user perception and feedback about the LMA, and it was relatively easy for us to obtain the support of university students and collect valid sample data.
3. As the main users of LMAs, the university students’ use experience and feedback will improve LMA information services and promote the development of LMAs.
PEOU, PU, and BI are the core variables of TAM, as proposed by Davis [22]. SI and FCs are the core variables in UTAUT, as proposed by Venkatesh et al. [25]. TAM and UTAUT are widely used to study user adoption of information systems and technology and have been proved to be effective. Based on mature scales described in the literature [22,25] and the specific characteristics of LMAs, we designed measurement items for each construct. PEOU, PU, and BI were adapted from Davis [22], whereas SI and FCs were adapted from Venkatesh et al. [25]. Detailed information about the constructs is shown in Table 1.
The data used to test the research model were collected from the responses to a questionnaire. Each item was scored on a five-point Likert scale ranging from 1 (strongly disagree) to 5 (strongly agree). To avoid semantic problems, we improved the initial questionnaire according to suggestions from two experts and scholars in library and information science. Then, to examine the validity of the questionnaire, we administered it in a pretest to 15 students, and we revised it according to their suggestions.
The formal paper questionnaire asked for the respondents’ demographic information and had questions based on the five-point Likert scale. With the help of library directors and their staff, we targeted student groups and students taking information retrieval courses at three universities in Wuhan, all of which had developed LMAs and offered information retrieval courses in the first semester of junior year. Members of the research group explained the purpose and significance of the survey to the respondents to garner their support. Each participant had a smartphone that supported the use of an LMA and had used the LMA. First, they were asked to download the Chaoxing LMA, and then use it to find content related to their course. The questionnaire was given to them at their next class to get their feedback. Students who were interested in participating in future research were encouraged to leave their contact information at the end of the questionnaire. We first distributed and collected the questionnaire during the period September 20–27, 2017 (T1), receiving 252 valid responses. We did the same again during December 20–27, 2017 (T2), receiving 226 valid responses. The other 26 responses at T2 were invalid, so that we had 226 pairs of valid responses. The interval between the two questionnaires was three months. The course teacher was not present when the questionnaire was distributed or collected. We analyzed the 226 pairs of valid responses to identify the changes in the user perception of the LMA during those three months. Table 2 presents the demographic data of the 226 respondents, of whom 59.3% were male, 40.7% were female, 88.9% were under 25 years, 84.5% were undergraduate students, and 77.4% were majoring in human and social sciences.
We used partial least-squares structural equation modeling (PLS-SEM) in the empirical analysis, based on the following main considerations: (i) PLS-SEM is suitable for exploratory research, (ii) the objective of the present study was to explain and predict target constructs, and (iii) the sample was small [26,27].
6.1 Analysis of Measurement Model
To assess the reliability and validity of the variables in the research model, an internal-consistency reliability test and a convergent validity test were performed using SmartPLS 2.0. The results are shown in Tables 3 and 4. The internal-consistency reliability was measured with the composite reliability (CR), a metric suggested by Fornell et al. [28]. The convergent validity was measured with the average variance extracted (AVE) of the potential variables and the corresponding factor loading. The Cronbach’s α of each construct was also calculated. Table 3 shows that all the CR values were above 0.7969 and that all the Cronbach’s α values were above 0.6648, suggesting that the reliability of the questionnaire data is high. The AVE values were above 0.50, indicating good convergent validity, as suggested by Fornell et al. [28]. For satisfactory discriminant validity, the square root of the AVE from the construct should be larger than the correlation shared between the construct and other constructs in the mode. As shown in Table 4, the square root of the AVE for each construct is larger than its corresponding correlation coefficients with other constructs, satisfying Fornell et al. criteria for discriminant validity [28].
6.2 Analysis of Structural Model
We estimated the structural model using SmartPLS 2.0. The results are presented in Fig. 2 and Table 5. The significance values of all paths are at the levels 0.001, 0.01, or 0.05. SI directly influences PU and BI, FCs influence PEOU and BI, and PEOU influences PU. Furthermore, the core variables of TAM (PU and PEOU) influence users’ BI to use the LMA. The results from SmartPLS show the significant effects of all the factors used in this study.
Figure 2: Results of the structural equation model
According to Table 5, hypotheses H1a–H7a are supported. At time point T1, SI had positive significant effects on PU and BI (H5a: path coefficient β = 0.2091, p < 0.001; H4a: β = 0.0807, p < 0.001), and FCs had powerful influences on BI and PEOU (H6a: β = 0.0017, p < 0.05; H7a: β = 0.4418, p < 0.001). PEOU had effects on PU and BI (H3a: β = 0.5115, p < 0.001; H1a: β = 0.4970, p < 0.001), and PU had a positive and significant influence on BI (H2a: β = 0.2821, p < 0.001). At time point T2, SI had positive significant effects on PU and BI (H5a: β = 0.1300, p < 0.001; H4a: β = 0.0763, p < 0.01), and FCs had powerful influences on BI and PEOU (H6a: β = 0.2325, p < 0.05; H7a: β = 0.6056, p < 0.001). PEOU had effects on PU and BI (H3a: β = 0.4685, p < 0.001; H1a: β = 0.1207, p < 0.001), and PU had a positive and significant influence on BI (H2a: β = 0.2952, p < 0.001).
According to Table 5, hypotheses H1b–H7b are also supported. Comparing T2 with T1, the impacts of PU and FCs on users’ BI to use the LMA were enhanced, as was the impact of FCs on the PEOU. However, the impacts of PEOU and SI on users’ BI to use the LMA were weaker, as were the impacts of SI and PEOU on PU.
At T1, R2 was 0.5498 for BI (namely the explanatory power of the model variables was 0.5498 for BI), 0.1951 for PEOU, and 0.3879 for PU. However, at T2, R2 was 0.3245 for BI, 0.3667 for PEOU, and 0.2729 for PU. Therefore, as use time increases, the explanatory power of the model variables for users’ BI to use the LMA decreased, which indicates that with accumulating experience, whether a user decides to continue using the LMA depends on elements other than PEOU, PU, SI, or FCs.
The results of the significance analysis of the path coefficients show that PU, PEOU, SI, and FCs affect the UPB of the LMA and that the strengths of the influencing factors and their significance to user BI differed at time points T1 and T2. Below, we discuss some meaningful findings.
7.1 With Increasing Use Time, TAM Remains Universal for UPB of Library Mobile Apps
At time points T1 and T2, hypotheses H1a, H2a, and H3a were supported, consistent with the work of Davis [22]. Furthermore, the present study supports the ability of TAM to predict and interpret users’ BI toward the LMA. In the present study, PEOU and PU positively affect users’ BI to use the LMA. Users initially accepted and used it, and they often attached greater consideration to the PEOU and PU of the LMA because it was new to them. If individuals perceive that an LMA is easy to operate and useful for their learning and working, then they will be more likely to use it. Also, easy operation will enhance the users’ experiences of using an LMA, thereby enhancing its PU.
7.2 With Increasing Use Time, the Additional Variables also Affected Users’ BI
SI has a positive effect on users’ BI to use the LMA. SI positively affects the PU of the LMA, and the present results are consistent with those of Wang et al. [9]. Users are affected by publicity from their school and the recommendations of friends, teachers, and others, all of which enhance PU so that someone will be more inclined to use the LMA. FCs positively affect users’ BI to use the LMA, and the present results are consistent with those of Ming et al. [14]. FCs, such as a free and stable mobile network and access to a mobile device, positively affect PEOU, because the LMA requires a mobile device connected to a mobile network.
7.3 UPB has Dynamic Differences
Comparing T2 with T1, the empirical results show that PU and FCs have an increased impact on users’ BI to use the LMA, and FCs has an increased impact on PEOU. The impacts of PEOU and SI on users’ BI to use the LMA became weaker, as did the impacts of SI and PEOU on PU.
For college students who are accustomed to using various applications, PEOU has a significant influence on BI in the early stage of use. However, with increasing use time, the impacts of PEOU on PU and BI gradually diminish. PU reflects the degree to which the value of an LMA aligns with the person’s needs. With the accumulation of use experience, if users think that the LMA can meet their needs well and that using it can significantly improve their efficiency, then the impact of PU on actual use will increase. In the early stage of use, users are susceptible to the behavior and comments of others. Therefore, SI has a great influence on someone’s initial use behavior. As use time increases, the use experience becomes enhanced, the user becomes more rational, the impact of SI on PU weakens, and the person pays more attention to the actual utility and convenience of the LMA. Moreover, LMAs rely on the speed, stability, and coverage of mobile networks. Thus, the status of the mobile network in a college has a direct impact on students’ attitudes to the LMA. After initial use, the impact of FCs on PEOU gradually weakens. Because users are increasingly dependent on being connected to a high-speed and stable mobile network, the impact of network quality on continuous use increases, and the impact of FCs on users’ BI to use the LMA increases [29].
First, we will consider adding more variables that affect UPB—such as information quality, service quality, and system quality—to extend and improve the model so that it better predicts and explains users’ dynamic behavior toward an LMA. Second, we will further explore the influencing factors for different user populations and users’ dynamic perception of an LMA. Third, we will expand the inclusion criteria for participants. By using artificial intelligence to analyze the log data produced by an LMA, we may be better able to assess users’ dynamic behavior and may be able to verify the dynamic applicability of our conclusions by dynamically tracking the perception and feedback of users at different times. This would allow us to compare and analyze the influencing factors of LMAs and the differences in user behavior in different regions [30].
Under the background of the increasingly intelligent modern library and the deep learning of users, this paper puts forward the problem of how to improve the service quality of LMA and reduce the loss rate of users. From a dynamic perspective, we constructed a UPB model of LMAs based on TAM, and introduced SI and FC. Then we distributed questionnaires to collect first-hand user data at two different time points, and conducted an empirical analysis using SmartPLS 2.0. The empirical results showed that with increasing use time, TAM remains universal for UPB of LMAs, extended variables, SI and FC, affect users’ BI, and the factors that affect users’ BI to use LMAs are different at different times. With increasing use time, PU and FC have more effect on users’ BI to use LMAs, and the impacts of SI and PEOU diminish. In the future, we can have a deep understanding of the dynamic behavior of users in the use process and optimize the use process for designers and developers from the perspective of users.
Acknowledgement: We thank the anonymous referees for their useful ideas.
Funding Statement: The authors received no specific funding for this study.
Conflicts of Interest: The authors declare that they have no conflicts of interest to report regarding the present study.
References
1. X. Q. Zhang, “Problems and countermeasures of domestic library mobile services: Based on the investigation and analysis of project 985 library mobile service platforms,” Information Studies: Theory & Application, vol. 41, no. 11, pp. 73–78, 2018. [Google Scholar]
2. T. C. Xu, Y. T. Pan, T. T. Feng, X. Y. Yang, Q. Y. Wei et al., “Research on current situation and development strategy of mobile library services mode in colleges and universities,” Library and Information Service, vol. 64, no. 3, pp. 71–82, 2020. [Google Scholar]
3. Q. Y. Wei, F. Yuan, H. Jia, R. Huo and G. N. Hou, “Mobile library service of the National Library of China and the provincial public libraries,” Journal of Library Science in China, vol. 40, no. 3, pp. 50–63, 2014. [Google Scholar]
4. J. Jasperson, P. E. Carter and R. W. Zmud, “A comprehensive conceptualization of post-adoptive behaviors associated with information technology enabled work systems,” MIS Quarterly, vol. 29, no. 3, pp. 525–557, 2005. [Google Scholar]
5. J. Hey, J. S. Sandhu, C. Newman, J. Hsu, C. Danies et al., “Designing mobile digital library services for pre-engineering and technology literacy,” International Journal of Engineering Education, vol. 23, no. 3, pp. 441–453, 2007. [Google Scholar]
6. I. Pasanen, “Around the world to Helsinki university of technology: New library services for mobile users,” Library Hi Tech News, vol. 19, no. 5, pp. 25–27, 2002. [Google Scholar]
7. E. Kroski, “On the move with the mobile web: Libraries and mobile technologies,” Library Technology Reports, vol. 44, no. 5, pp. 1–48, 2008. [Google Scholar]
8. J. S. Zhang, Y. C. Sun, L. J. Zhu and X. D. Qiao, “A synergetic mechanism for digital library service in mobile and cloud computing environment,” Personal and Ubiquitous Computing, vol. 18, no. 8, pp. 1845–1854, 2014. [Google Scholar]
9. C. Y. Wang, H. R. Ke and W. C. Lu, “Design and performance evaluation of mobile web services in libraries: A case study of the Oriental Institute of Technology Library,” Electronic Library, vol. 30, no. 1, pp. 33–50, 2012. [Google Scholar]
10. D. Tian, “Analysis of status of mobile library APP in China,” Journal of the National Library of China, vol. 24, no. 5, pp. 74–80, 2015. [Google Scholar]
11. Y. F. Huang and L. R. Yang, “An empirical study on mobile library user’s acceptance model,” Modern Information, vol. 33, no. 11, pp. 172–177, 2013. [Google Scholar]
12. J. Al Mursalin, “Information system adoption and usage: Validating UTAUT model for Bangladeshi SMES,” International Journal of Management, IT and Engineering, vol. 2, no. 10, pp. 1–19, 2012. [Google Scholar]
13. S. Wang, “Research on user acceptance model of the mobile library,” Information Science, vol. 31, no. 4, pp. 39–44, 2013. [Google Scholar]
14. J. R. Ming and C. Q. Guo, “Construction of behavioral model and empirical study of user adoption of library mobile applications,” Library Tribune, vol. 38, no. 10, pp. 93–101, 2018. [Google Scholar]
15. M. Jaradat, “Exploring the factors that affect intention to use mobile phones in Jordanian academic library,” International Journal of Information Technology and Web Engineering, vol. 7, no. 4, pp. 34–49, 2012. [Google Scholar]
16. N. Aharony, “Mobile libraries: Librarians’ and students’ perspectives,” College & Research Libraries, vol. 75, no. 2, pp. 202–217, 2014. [Google Scholar]
17. S. J. Saravani and G. Haddow, “The mobile library and staff preparedness: Exploring staff competencies using the unified theory of acceptance and use of technology model,” Australian Academic & Research Libraries, vol. 42, no. 3, pp. 179–190, 2011. [Google Scholar]
18. W. T. Wang, Y. Q. Xie and J. Li, “A study on college students’ intention of using function-integrated mobile library,” Library Tribune, vol. 34, no. 11, pp. 73–80, 2014. [Google Scholar]
19. S. Vongjaturapat, S. Chabeesuk, N. Chotikakamthorn and S. Tongkhambanchong, “Analysis of factor influencing the tablet acceptance for library information services: A combination of UTAUT and TTF model,” Journal of Information & Knowledge Management, vol. 14, no. 3, pp. 1–23, 2015. [Google Scholar]
20. M. Zhang, X. Shen, M. Zhu and J. Yang, “Which platform should I choose? Factors influencing consumers’ channel transfer intention from web-based to mobile library service,” Library Hi Tech, vol. 34, no. 1, pp. 2–20, 2016. [Google Scholar]
21. J. R. Ming, C. Q. Guo and J. Zhang, “A research overview of user perception behavior for Chinese mobile library based on technology acceptance model,” Library Tribune, vol. 37, no. 7, pp. 125–131, 2017. [Google Scholar]
22. F. D. Davis, “Perceived usefulness, perceived ease of use, and user acceptance of information technology,” MIS Quarterly, vol. 13, no. 3, pp. 319–340, 1989. [Google Scholar]
23. S. S. Liaw and H. M. Huang, “An investigation of user attitudes toward search engines as an information retrieval tool,” Computers in Human Behavior, vol. 19, no. 6, pp. 751–765, 2003. [Google Scholar]
24. G. C. Huang and A. Kumar, “Explaining consumer acceptance of handheld internet devices,” Journal of Business Research, vol. 58, no. 5, pp. 553–558, 2005. [Google Scholar]
25. V. Venkatesh, M. G. Morris, G. B. Davis and F. D. Davis, “User acceptance of information technology: Toward a unified view,” MIS Quarterly, vol. 27, no. 3, pp. 425–478, 2003. [Google Scholar]
26. M. Sarstedt, C. M. Ringle and J. F. Hair, “Partial least squares structural equation modeling,” Handbook of Market Research, vol. 26, no. 1, pp. 1–40, 2017. [Google Scholar]
27. J. Refugio Romo-Gonzalez, J. Tarango and J. D. Machin-Mastromatteo, “PLS SEM, a quantitative methodology to test theoretical models from library and information science,” Information Development, vol. 34, no. 5, pp. 526–531, 2018. [Google Scholar]
28. C. Fornell and D. F. Larcker, “Structural equation models with unobservable variables and measurement errors,” Journal of Marketing Research, vol. 18, no. 3, pp. 382–388, 1981. [Google Scholar]
29. Y. Sun, Y. Ding, J. Jiang and V. G. Duffy, “Measuring mental workload using ERPs based on FIR, ICA, and MARA,” Computer Systems Science and Engineering, vol. 41, no. 2, pp. 781–794, 2022. [Google Scholar]
30. D. Dangi, A. Bhagat and D. K. Dixit, “Emerging applications of artificial intelligence, machine learning and data science,” Computers, Materials & Continua, vol. 70, no. 3, pp. 5399–5419, 2022. [Google Scholar]
Cite This Article
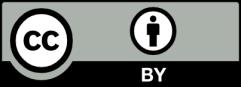