Open Access
ARTICLE
Visual Object Tracking Based on Modified LeNet-5 and RCCF
Department of Computer Science and Engineering, Koneru Lakshmaiah Education Foundation, Deemed to be University, Hyderabad, 500075, Telangana, India
* Corresponding Author: Barnali Gupta Banik. Email:
Computer Systems Science and Engineering 2023, 46(1), 1127-1139. https://doi.org/10.32604/csse.2023.032904
Received 01 June 2022; Accepted 29 September 2022; Issue published 20 January 2023
Abstract
The field of object tracking has recently made significant progress. Particularly, the performance results in both deep learning and correlation filters, based trackers achieved effective tracking performance. Moreover, there are still some difficulties with object tracking for example illumination and deformation (DEF). The precision and accuracy of tracking algorithms suffer from the effects of such occurrences. For this situation, finding a solution is important. This research proposes a new tracking algorithm to handle this problem. The features are extracted by using Modified LeNet-5, and the precision and accuracy are improved by developing the Real-Time Cross-modality Correlation Filtering method (RCCF). In Modified LeNet-5, the visual tracking performance is improved by adjusting the number and size of the convolution kernels in the pooling and convolution layers. The high-level, middle-level, and handcraft features are extracted from the modified LeNet-5 network. The handcraft features are used to determine the specific location of the target because the handcraft features contain more spatial information regarding the visual object. The LeNet features are more suitable for a target appearance change in object tracking. Extensive experiments were conducted by the Object Tracking Benchmarking (OTB) databases like OTB50 and OTB100. The experimental results reveal that the proposed tracker outperforms other state-of-the-art trackers under different problems. The experimental simulation is carried out in python. The overall success rate and precision of the proposed algorithm are 93.8% and 92.5%. The average running frame rate reaches 42 frames per second, which can meet the real-time requirements.Keywords
Cite This Article
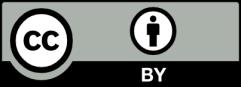
This work is licensed under a Creative Commons Attribution 4.0 International License , which permits unrestricted use, distribution, and reproduction in any medium, provided the original work is properly cited.