Open Access
ARTICLE
Dynamic Analogical Association Algorithm Based on Manifold Matching for Few-Shot Learning
1 Chengdu Institute of Computer Applications, Chinese Academy of Sciences, Chengdu, 610041, China
2 University of Chinese Academy of Sciences, Beijing, 100049, China
* Corresponding Author: Xiaolin Qin. Email:
Computer Systems Science and Engineering 2023, 46(1), 1233-1247. https://doi.org/10.32604/csse.2023.032633
Received 24 May 2022; Accepted 12 July 2022; Issue published 20 January 2023
Abstract
At present, deep learning has been well applied in many fields. However, due to the high complexity of hypothesis space, numerous training samples are usually required to ensure the reliability of minimizing experience risk. Therefore, training a classifier with a small number of training examples is a challenging task. From a biological point of view, based on the assumption that rich prior knowledge and analogical association should enable human beings to quickly distinguish novel things from a few or even one example, we proposed a dynamic analogical association algorithm to make the model use only a few labeled samples for classification. To be specific, the algorithm search for knowledge structures similar to existing tasks in prior knowledge based on manifold matching, and combine sampling distributions to generate offsets instead of two sample points, thereby ensuring high confidence and significant contribution to the classification. The comparative results on two common benchmark datasets substantiate the superiority of the proposed method compared to existing data generation approaches for few-shot learning, and the effectiveness of the algorithm has been proved through ablation experiments.Keywords
Cite This Article
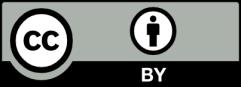
This work is licensed under a Creative Commons Attribution 4.0 International License , which permits unrestricted use, distribution, and reproduction in any medium, provided the original work is properly cited.