Open Access
ARTICLE
Deep Learning Framework for the Prediction of Childhood Medulloblastoma
1 Department of Biomedical Engineering, Veltech Rangarajan Dr. Sagunthala R&D Institute of Science and Technology, Chennai, Tamil Nadu, India
2 Department of Electronics and Instrumentation Engineering, Saveetha Engineering College, Chennai, Tamil Nadu, India
3 Department of Information Technology, J.J. College of Engineering and Technology, Trichy, Tamil Nadu, India
4 Department of Information Technology, R.M.D Engineering College, Kavaraipettai, Chennai, Tamil Nadu, India
* Corresponding Author: M. Muthalakshmi. Email:
Computer Systems Science and Engineering 2023, 46(1), 735-747. https://doi.org/10.32604/csse.2023.032449
Received 18 May 2022; Accepted 19 October 2022; Issue published 20 January 2023
Abstract
This research work develops new and better prognostic markers for predicting Childhood MedulloBlastoma (CMB) using a well-defined deep learning architecture. A deep learning architecture could be designed using ideas from image processing and neural networks to predict CMB using histopathological images. First, a convolution process transforms the histopathological image into deep features that uniquely describe it using different two-dimensional filters of various sizes. A 10-layer deep learning architecture is designed to extract deep features. The introduction of pooling layers in the architecture reduces the feature dimension. The extracted and dimension-reduced deep features from the arrangement of convolution layers and pooling layers are used to classify histopathological images using a neural network classifier. The performance of the CMB classification system is evaluated using 1414 (10× magnification) and 1071 (100× magnification) augmented histopathological images with five classes of CMB such as desmoplastic, nodular, large cell, classic, and normal. Experimental results show that the average classification accuracy of 99.38% (10×) and 99.07% (100×) is attained by the proposed CNB classification system.Keywords
Cite This Article
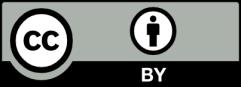
This work is licensed under a Creative Commons Attribution 4.0 International License , which permits unrestricted use, distribution, and reproduction in any medium, provided the original work is properly cited.