Open Access
ARTICLE
Detection of Abnormal Network Traffic Using Bidirectional Long Short-Term Memory
School of Information and Communication Technology, Hanoi University of Science and Technology, Hanoi, 10000, Vietnam
* Corresponding Author: Quang H. Nguyen. Email:
Computer Systems Science and Engineering 2023, 46(1), 491-504. https://doi.org/10.32604/csse.2023.032107
Received 06 May 2022; Accepted 28 October 2022; Issue published 20 January 2023
Abstract
Nowadays, web systems and servers are constantly at great risk from cyberattacks. This paper proposes a novel approach to detecting abnormal network traffic using a bidirectional long short-term memory (LSTM) network in combination with the ensemble learning technique. First, the binary classification module was used to detect the current abnormal flow. Then, the abnormal flows were fed into the multilayer classification module to identify the specific type of flow. In this research, a deep learning bidirectional LSTM model, in combination with the convolutional neural network and attention technique, was deployed to identify a specific attack. To solve the real-time intrusion-detecting problem, a stacking ensemble-learning model was deployed to detect abnormal intrusion before being transferred to the attack classification module. The class-weight technique was applied to overcome the data imbalance between the attack layers. The results showed that our approach gained good performance and the F1 accuracy on the CICIDS2017 data set reached 99.97%, which is higher than the results obtained in other research.Keywords
Cite This Article
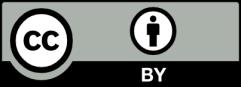