Open Access
ARTICLE
Eye Detection-Based Deep Belief Neural Networks and Speeded-Up Robust Feature Algorithm
1 Faculty of Computers and Information, Mansoura University, Mansoura, 35516, Egypt
2 Deanship of Scientific Research, Umm Al-Qura University, Makkah, 21955, KSA
3 Delta Higher Institute of Engineering and Technology, Mansoura, 35111, Egypt
4 Faculty of Artificial Intelligence, Kafrelsheikh University, Kafrelsheikh, 33511, Egypt
* Corresponding Author: Samaa M. Shohieb. Email:
Computer Systems Science and Engineering 2023, 45(3), 3195-3213. https://doi.org/10.32604/csse.2023.034092
Received 06 July 2022; Accepted 22 September 2022; Issue published 21 December 2022
Abstract
The ability to detect and localize the human eye is critical for use in security applications and human identification and verification systems. This is because eye recognition algorithms have multiple challenges, such as multi-pose variations, ocular parts, and illumination. Moreover, the modern security applications fail to detect facial expressions from eye images. In this paper, a Speeded-Up Roust Feature (SURF) Algorithm was utilized to localize the face images of the enrolled subjects. We highlighted on eye and pupil parts to be detected based on SURF, Hough Circle Transform (HCT), and Local Binary Pattern (LBP). Afterward, Deep Belief Neural Networks (DBNN) were used to classify the input features results from the SURF algorithm. We further determined the correctly and wrongly classified subjects using a confusion matrix with two class labels to classify people whose eye images are correctly detected. We apply Stochastic Gradient Descent (SGD) optimizer to address the overfitting problem, and the hyper-parameters are fine-tuned based on the applied DBNN. The accuracy of the proposed system is determined based on SURF, LBP, and DBNN classifier achieved 95.54% for the ORL dataset, 94.07% for the BioID, and 96.20% for the CASIA-V5 dataset. The proposed approach is more reliable and more advanced when compared with state-of-the-art algorithms.Keywords
Cite This Article
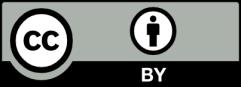