Open Access
ARTICLE
Enhanced Gorilla Troops Optimizer with Deep Learning Enabled Cybersecurity Threat Detection
1 Department of Information Systems, College of Computer and Information Sciences, Princess Nourah bint Abdulrahman University, P.O. Box 84428, Riyadh, 11671, Saudi Arabia
2 Prince Saud Al Faisal Institute for Diplomatic Studies, Riyadh, Saudi Arabia
3 Department of Industrial Engineering, College of Engineering at Alqunfudah, Umm Al-Qura University, Saudi Arabia
4 Department of Information Technology, College of Computers and Information Technology, Taif University, P.O. Box 11099, Taif, 21944, Saudi Arabia
5 Department of Information Systems, College of Computer and Information Sciences, King Saud University, Saudi Arabia
6 Department of Computer Science, College of Computers and Information Technology, University of Bisha, Saudi Arabia
7 Department of Computer Science, Faculty of Computers and Information Technology, Future University in Egypt, New Cairo, 11835, Egypt
8 Department of Computer and Self Development, Preparatory Year Deanship, Prince Sattam bin Abdulaziz University, AlKharj, Saudi Arabia
* Corresponding Author: Manar Ahmed Hamza. Email:
Computer Systems Science and Engineering 2023, 45(3), 3037-3052. https://doi.org/10.32604/csse.2023.033970
Received 02 July 2022; Accepted 18 August 2022; Issue published 21 December 2022
Abstract
Recent developments in computer networks and Internet of Things (IoT) have enabled easy access to data. But the government and business sectors face several difficulties in resolving cybersecurity network issues, like novel attacks, hackers, internet criminals, and so on. Presently, malware attacks and software piracy pose serious risks in compromising the security of IoT. They can steal confidential data which results in financial and reputational losses. The advent of machine learning (ML) and deep learning (DL) models has been employed to accomplish security in the IoT cloud environment. This article presents an Enhanced Artificial Gorilla Troops Optimizer with Deep Learning Enabled Cybersecurity Threat Detection (EAGTODL-CTD) in IoT Cloud Networks. The presented EAGTODL-CTD model encompasses the identification of the threats in the IoT cloud environment. The proposed EAGTODL-CTD model mainly focuses on the conversion of input binary files to color images, where the malware can be detected using an image classification problem. The EAGTODL-CTD model pre-processes the input data to transform to a compatible format. For threat detection and classification, cascaded gated recurrent unit (CGRU) model is exploited to determine class labels. Finally, EAGTO approach is employed as a hyperparameter optimizer to tune the CGRU parameters, showing the novelty of our work. The performance evaluation of the EAGTODL-CTD model is assessed on a dataset comprising two class labels namely malignant and benign. The experimental values reported the supremacy of the EAGTODL-CTD model with increased accuracy of 99.47%.Keywords
Cite This Article
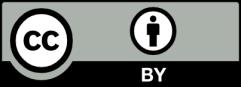
This work is licensed under a Creative Commons Attribution 4.0 International License , which permits unrestricted use, distribution, and reproduction in any medium, provided the original work is properly cited.