Open Access
ARTICLE
3D Path Optimisation of Unmanned Aerial Vehicles Using Q Learning-Controlled GWO-AOA
1 Department of Instrumentation and Control Engineering, Dr. B. R. Ambedkar National Institute of Technology Jalandhar, Punjab, 144027, India
2 Department of Industrial and Production Engineering, Dr. B. R. Ambedkar National Institute of Technology Jalandhar, Punjab, 144027, India
3 Department of Electronics and Communication Engineering, Zagazig University, Zagazig, Sharqia, 44519, Egypt
4 Department of Information Technology, College of Computer and Information Sciences, Princess Nourah bint Abdulrahman University, Riyadh, 84428, Saudi Arabia
* Corresponding Author: Naglaa F. Soliman. Email:
Computer Systems Science and Engineering 2023, 45(3), 2483-2503. https://doi.org/10.32604/csse.2023.032737
Received 28 May 2022; Accepted 30 June 2022; Issue published 21 December 2022
Abstract
Unmanned Aerial Vehicles (UAVs) or drones introduced for military applications are gaining popularity in several other fields as well such as security and surveillance, due to their ability to perform repetitive and tedious tasks in hazardous environments. Their increased demand created the requirement for enabling the UAVs to traverse independently through the Three Dimensional (3D) flight environment consisting of various obstacles which have been efficiently addressed by metaheuristics in past literature. However, not a single optimization algorithms can solve all kind of optimization problem effectively. Therefore, there is dire need to integrate metaheuristic for general acceptability. To address this issue, in this paper, a novel reinforcement learning controlled Grey Wolf Optimisation-Archimedes Optimisation Algorithm (QGA) has been exhaustively introduced and exhaustively validated firstly on 22 benchmark functions and then, utilized to obtain the optimum flyable path without collision for UAVs in three dimensional environment. The performance of the developed QGA has been compared against the various metaheuristics. The simulation experimental results reveal that the QGA algorithm acquire a feasible and effective flyable path more efficiently in complicated environment.Keywords
Cite This Article
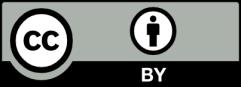
This work is licensed under a Creative Commons Attribution 4.0 International License , which permits unrestricted use, distribution, and reproduction in any medium, provided the original work is properly cited.