Open Access
ARTICLE
Human Stress Recognition by Correlating Vision and EEG Data
SRM Institute of Science and Technology, Department of Data Science and Business Systems, School of Computing, Kattankulathur, 603203, Tamilnadu, India
* Corresponding Author: S. Praveenkumar. Email:
Computer Systems Science and Engineering 2023, 45(3), 2417-2433. https://doi.org/10.32604/csse.2023.032480
Received 19 May 2022; Accepted 29 June 2022; Issue published 21 December 2022
Abstract
Because stress has such a powerful impact on human health, we must be able to identify it automatically in our everyday lives. The human activity recognition (HAR) system use data from several kinds of sensors to try to recognize and evaluate human actions automatically recognize and evaluate human actions. Using the multimodal dataset DEAP (Database for Emotion Analysis using Physiological Signals), this paper presents deep learning (DL) technique for effectively detecting human stress. The combination of vision-based and sensor-based approaches for recognizing human stress will help us achieve the increased efficiency of current stress recognition systems and predict probable actions in advance of when fatal. Based on visual and EEG (Electroencephalogram) data, this research aims to enhance the performance and extract the dominating characteristics of stress detection. For the stress identification test, we utilized the DEAP dataset, which included video and EEG data. We also demonstrate that combining video and EEG characteristics may increase overall performance, with the suggested stochastic features providing the most accurate results. In the first step, CNN (Convolutional Neural Network) extracts feature vectors from video frames and EEG data. Feature Level (FL) fusion that combines the features extracted from video and EEG data. We use XGBoost as our classifier model to predict stress, and we put it into action. The stress recognition accuracy of the proposed method is compared to existing methods of Decision Tree (DT), Random Forest (RF), AdaBoost, Linear Discriminant Analysis (LDA), and K-Nearest Neighborhood (KNN). When we compared our technique to existing state-of-the-art approaches, we found that the suggested DL methodology combining multimodal and heterogeneous inputs may improve stress identification.Keywords
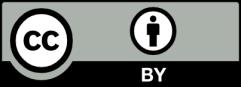