Open Access
ARTICLE
Multi-label Emotion Classification of COVID–19 Tweets with Deep Learning and Topic Modelling
1 Department of Information Technology, Sri Sai Ram Institute of Technology, Chennai, Tamilnadu, India
2 Department of Computer Science and Engineering, Sethu Institute of Technology, Madurai, Tamilnadu, India
* Corresponding Author: K. Anuratha. Email:
Computer Systems Science and Engineering 2023, 45(3), 3005-3021. https://doi.org/10.32604/csse.2023.031553
Received 21 April 2022; Accepted 27 August 2022; Issue published 21 December 2022
Abstract
The COVID-19 pandemic has become one of the severe diseases in recent years. As it majorly affects the common livelihood of people across the universe, it is essential for administrators and healthcare professionals to be aware of the views of the community so as to monitor the severity of the spread of the outbreak. The public opinions are been shared enormously in microblogging media like twitter and is considered as one of the popular sources to collect public opinions in any topic like politics, sports, entertainment etc., This work presents a combination of Intensity Based Emotion Classification Convolution Neural Network (IBEC-CNN) model and Non-negative Matrix Factorization (NMF) for detecting and analyzing the different topics discussed in the COVID-19 tweets as well the intensity of the emotional content of those tweets. The topics were identified using NMF and the emotions are classified using pretrained IBEC-CNN, based on predefined intensity scores. The research aimed at identifying the emotions in the Indian tweets related to COVID-19 and producing a list of topics discussed by the users during the COVID-19 pandemic. Using the Twitter Application Programming Interface (Twitter API), huge numbers of COVID-19 tweets are retrieved during January and July 2020. The extracted tweets are analyzed for emotions fear, joy, sadness and trust with proposed Intensity Based Emotion Classification Convolution Neural Network (IBEC-CNN) model which is pretrained. The classified tweets are given an intensity score varies from 1 to 3, with 1 being low intensity for the emotion, 2 being the moderate and 3 being the high intensity. To identify the topics in the tweets and the themes of those topics, Non-negative Matrix Factorization (NMF) has been employed. Analysis of emotions of COVID-19 tweets has identified, that the count of positive tweets is more than that of count of negative tweets during the period considered and the negative tweets related to COVID-19 is less than 5%. Also, more than 75% negative tweets expressed sadness, fear are of low intensity. A qualitative analysis has also been conducted and the topics detected are grouped into themes such as economic impacts, case reports, treatments, entertainment and vaccination. The results of analysis show that the issues related to the pandemic are expressed different emotions in twitter which helps in interpreting the public insights during the pandemic and these results are beneficial for planning the dissemination of factual health statistics to build the trust of the people. The performance comparison shows that the proposed IBEC-CNN model outperforms the conventional models and achieved 83.71% accuracy. The % of COVID-19 tweets that discussed the different topics vary from 7.45% to 26.43% on topics economy, Statistics on cases, Government/Politics, Entertainment, Lockdown, Treatments and Virtual Events. The least number of tweets discussed on politics/government on the other hand the tweets discussed most about treatments.Keywords
Cite This Article
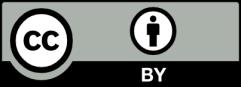
This work is licensed under a Creative Commons Attribution 4.0 International License , which permits unrestricted use, distribution, and reproduction in any medium, provided the original work is properly cited.