Open Access
ARTICLE
Feature Matching Combining Variable Velocity Model with Reverse Optical Flow
1 College of Automation, Nanjing University of Information Science & Technology, Nanjing, 210044, China
2 Wuxi Research Institute, Nanjing University of Information Science & Technology, Wuxi, 214100, China
3 Jiangsu Collaborative Innovation Center on Atmospheric Environment and Equipment Technology, Nanjing University of Information Science & Technology, Nanjing, 210044, China
4 Department of Civil and Environmental Engineering, Rensselaer Polytechnic Institute, Troy, 12180, NY, USA
* Corresponding Author: Wei Sun. Email:
Computer Systems Science and Engineering 2023, 45(2), 1083-1094. https://doi.org/10.32604/csse.2023.032786
Received 29 May 2022; Accepted 01 July 2022; Issue published 03 November 2022
Abstract
The ORB-SLAM2 based on the constant velocity model is difficult to determine the search window of the reprojection of map points when the objects are in variable velocity motion, which leads to a false matching, with an inaccurate pose estimation or failed tracking. To address the challenge above, a new method of feature point matching is proposed in this paper, which combines the variable velocity model with the reverse optical flow method. First, the constant velocity model is extended to a new variable velocity model, and the expanded variable velocity model is used to provide the initial pixel shifting for the reverse optical flow method. Then the search range of feature points is accurately determined according to the results of the reverse optical flow method, thereby improving the accuracy and reliability of feature matching, with strengthened interframe tracking effects. Finally, we tested on TUM data set based on the RGB-D camera. Experimental results show that this method can reduce the probability of tracking failure and improve localization accuracy on SLAM (Simultaneous Localization and Mapping) systems. Compared with the traditional ORB-SLAM2, the test error of this method on each sequence in the TUM data set is significantly reduced, and the root mean square error is only 63.8% of the original system under the optimal condition.Keywords
Cite This Article
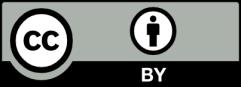
This work is licensed under a Creative Commons Attribution 4.0 International License , which permits unrestricted use, distribution, and reproduction in any medium, provided the original work is properly cited.