Open Access
ARTICLE
De-Noising Brain MRI Images by Mixing Concatenation and Residual Learning (MCR)
1 Department of Computer Science, Faculty of Information Technology, University of Central Punjab Lahore, Lahore, 54000, Pakistan
2 Department of Computer Science, COMSAT University Islamabad, Lahore Campus, Lahore, 54000, Pakistan
3 Faculty of Computers and Information Technology, Computer Science Department, University of Tabuk, Tabuk, 47711, Saudi Arabia
* Corresponding Author: Kazim Ali. Email:
Computer Systems Science and Engineering 2023, 45(2), 1167-1186. https://doi.org/10.32604/csse.2023.032508
Received 20 May 2022; Accepted 21 June 2022; Issue published 03 November 2022
Abstract
Brain magnetic resonance images (MRI) are used to diagnose the different diseases of the brain, such as swelling and tumor detection. The quality of the brain MR images is degraded by different noises, usually salt & pepper and Gaussian noises, which are added to the MR images during the acquisition process. In the presence of these noises, medical experts are facing problems in diagnosing diseases from noisy brain MR images. Therefore, we have proposed a de-noising method by mixing concatenation, and residual deep learning techniques called the MCR de-noising method. Our proposed MCR method is to eliminate salt & pepper and gaussian noises as much as possible from the brain MRI images. The MCR method has been trained and tested on the noise quantity levels 2% to 20% for both salt & pepper and gaussian noise. The experiments have been done on publically available brain MRI image datasets, which can easily be accessible in the experiments and result section. The Structure Similarity Index Measure (SSIM) and Peak Signal-to-Noise Ratio (PSNR) calculate the similarity score between the denoised images by the proposed MCR method and the original clean images. Also, the Mean Squared Error (MSE) measures the error or difference between generated denoised and the original images. The proposed MCR de-noising method has a 0.9763 SSIM score, 84.3182 PSNR, and 0.0004 MSE for salt & pepper noise; similarly, 0.7402 SSIM score, 72.7601 PSNR, and 0.0041 MSE for Gaussian noise at the highest level of 20% noise. In the end, we have compared the MCR method with the state-of-the-art de-noising filters such as median and wiener de-noising filters.Keywords
Cite This Article
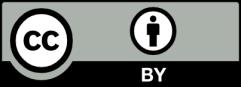
This work is licensed under a Creative Commons Attribution 4.0 International License , which permits unrestricted use, distribution, and reproduction in any medium, provided the original work is properly cited.