Open Access
ARTICLE
Brain Tumor: Hybrid Feature Extraction Based on UNet and 3DCNN
1 Center for System Design, Chennai Institute of Technology, Chennai, 600069, India
2 Department of Information Technology, Vel Tech Multi Tech Dr. Rangarajan Dr. Sakunthala Engineering College, Chennai, 600062, India
3 Department of Computer Science, College of Computer and Information Systems, Umm Al-Qura University, Makkah, 21955, Saudi Arabia
4 Department of Information Technology, College of Computers and Information Technology, Taif University, Taif, 21944, Saudi Arabia
* Corresponding Author: Tamilvizhi Thanarajan. Email:
Computer Systems Science and Engineering 2023, 45(2), 2093-2109. https://doi.org/10.32604/csse.2023.032488
Received 19 May 2022; Accepted 21 June 2022; Issue published 03 November 2022
Abstract
Automated segmentation of brain tumors using Magnetic Resonance Imaging (MRI) data is critical in the analysis and monitoring of disease development. As a result, gliomas are aggressive and diverse tumors that may be split into intra-tumoral groups by using effective and accurate segmentation methods. It is intended to extract characteristics from an image using the Gray Level Co-occurrence (GLC) matrix feature extraction method described in the proposed work. Using Convolutional Neural Networks (CNNs), which are commonly used in biomedical image segmentation, CNNs have significantly improved the precision of the state-of-the-art segmentation of a brain tumor. Using two segmentation networks, a U-Net and a 3D CNN, we present a major yet easy combinative technique that results in improved and more precise estimates. The U-Net and 3D CNN are used together in this study to get better and more accurate estimates of what is going on. Using the dataset, two models were developed and assessed to provide segmentation maps that differed fundamentally in terms of the segmented tumour sub-region. Then, the estimates was made by two separate models that were put together to produce the final prediction. In comparison to current state-of-the-art designs, the precision (percentage) was 98.35, 98.5, and 99.4 on the validation set for tumor core, enhanced tumor, and whole tumor, respectively.Keywords
Cite This Article
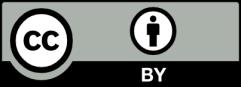
This work is licensed under a Creative Commons Attribution 4.0 International License , which permits unrestricted use, distribution, and reproduction in any medium, provided the original work is properly cited.