Open Access
ARTICLE
Hybrid of Distributed Cumulative Histograms and Classification Model for Attack Detection
1 Department of Electronics and Electrical Communications Engineering, Faculty of Electronic Engineering, Menoufia University, Menouf, 32952, Egypt
2 Alexandria Higher Institute of Engineering & Technology (AIET), Alexandria, Egypt
3 Department of Computer Science, Security Engineering Laboratory, Prince Sultan University, Riyadh, 11586, Saudi Arabia
4 Department of Information Technology, College of Computer and Information Sciences, Princess Nourah Bint Abdulrahman University, P.O. Box 84428, Riyadh, 11671, Saudi Arabia
5 Department of Computer Sciences, College of Computer and Information Sciences, Princess Nourah Bint Abdulrahman University, Riyadh, Saudi Arabia
* Corresponding Author: Hussah Nasser AlEisa. Email:
Computer Systems Science and Engineering 2023, 45(2), 2235-2247. https://doi.org/10.32604/csse.2023.032156
Received 09 May 2022; Accepted 10 June 2022; Issue published 03 November 2022
Abstract
Traditional security systems are exposed to many various attacks, which represents a major challenge for the spread of the Internet in the future. Innovative techniques have been suggested for detecting attacks using machine learning and deep learning. The significant advantage of deep learning is that it is highly efficient, but it needs a large training time with a lot of data. Therefore, in this paper, we present a new feature reduction strategy based on Distributed Cumulative Histograms (DCH) to distinguish between dataset features to locate the most effective features. Cumulative histograms assess the dataset instance patterns of the applied features to identify the most effective attributes that can significantly impact the classification results. Three different models for detecting attacks using Convolutional Neural Network (CNN) and Long Short-Term Memory Network (LSTM) are also proposed. The accuracy test of attack detection using the hybrid model was 98.96% on the UNSW-NP15 dataset. The proposed model is compared with wrapper-based and filter-based Feature Selection (FS) models. The proposed model reduced classification time and increased detection accuracy.Keywords
Cite This Article
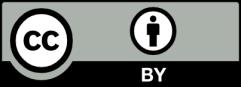