Open Access
ARTICLE
IoT-Deep Learning Based Activity Recommendation System
1 Department of Electronics and Communication Engineering, Nandha Engineering College, Perundurai, 638052, Tamilnadu, India
2 Department of Computer Science, Sona College of Arts and Science, Salem, 636005, Tamilnadu, India
3 Department of Artificial Intelligence and Machine Learning Engineering, Gokaraju Rangaraju Institute of Engineering and Technology, Hyderabad, 500090, Telangana, India
4 Department of Computer Science and Engineering, Kongu Engineering College, Perundurai, 638060, Tamilnadu, India
* Corresponding Author: Sharmilee Kannan. Email:
Computer Systems Science and Engineering 2023, 45(2), 2001-2016. https://doi.org/10.32604/csse.2023.031965
Received 02 May 2022; Accepted 08 June 2022; Issue published 03 November 2022
Abstract
The rising use of mobile technology and smart gadgets in the field of health has had a significant impact on the global community. Health professionals are increasingly making use of the benefits of these technologies, resulting in a major improvement in health care both in and out of clinical settings. The Internet of Things (IoT) is a new internet revolution that is a rising research area, particularly in health care. Healthcare Monitoring Systems (HMS) have progressed rapidly as the usage of Wearable Sensors (WS) and smartphones have increased. The existing framework of conventional telemedicine’s store-and-forward method has some issues, including the need for a nearby health centre with dedicated employees and medical devices to prepare patient reports. Patients’ health can be continuously monitored using advanced WS that can be fitted or embedded in their bodies. This research proposes an innovative and smart HMS, which is built using recent technologies such as the IoT and Machine Learning (ML). In this study, we present an innovative and intelligent HMS based on cutting-edge technologies such as the IoT and Deep Learning (DL) + Restricted Boltzmann Machine (RBM). This DL + RBM model is clever enough to detect and process a patient’s data using a medical Decision Support System (DSS) to determine whether the patient is suffering from a major health problem and treat it accordingly. The recommended system’s behavior is increasingly investigated using a cross-validation test that determines various demographically relevant standard measures. Through a healthcare DSS, this framework is clever enough to detect and analyze a patient’s data. Experiment results further reveal that the proposed system is efficient and clever enough to deliver health care. The data reported in this study demonstrate the notion. This device is a low-cost solution for people living in distant places; anyone can use it to determine if they have a major health problem and seek treatment by contacting nearby hospitals.Keywords
Cite This Article
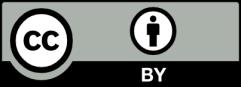
This work is licensed under a Creative Commons Attribution 4.0 International License , which permits unrestricted use, distribution, and reproduction in any medium, provided the original work is properly cited.