Open Access
ARTICLE
Dipper Throated Algorithm for Feature Selection and Classification in Electrocardiogram
1 Department of Computer Sciences, College of Computer and Information Sciences, Princess Nourah bint Abdulrahman University, P.O. Box 84428, Riyadh, 11671, Saudi Arabia
2 Department of Computer Science, Faculty of Computer and Information Sciences, Ain Shams University, 11566, Cairo, Egypt
3 Department of Computer Science, College of Computing and Information Technology, Shaqra University, 11961, Saudi Arabia
4 Computer Engineering and Control Systems Department, Faculty of Engineering, Mansoura University, Mansoura, 35516, Egypt
5 Electronics and Communications Engineering Department, Faculty of Engineering, Delta University for Science and Technology, Mansoura, Egypt
6 Department of Communications and Electronics, Delta Higher Institute of Engineering and Technology, Mansoura, 35111, Egypt
7 Faculty of Artificial Intelligence, Delta University for Science and Technology, Mansoura, 35712, Egypt
* Corresponding Author: Amel Ali Alhussan. Email:
Computer Systems Science and Engineering 2023, 45(2), 1469-1482. https://doi.org/10.32604/csse.2023.031943
Received 01 May 2022; Accepted 08 June 2022; Issue published 03 November 2022
Abstract
Arrhythmia has been classified using a variety of methods. Because of the dynamic nature of electrocardiogram (ECG) data, traditional handcrafted approaches are difficult to execute, making the machine learning (ML) solutions more appealing. Patients with cardiac arrhythmias can benefit from competent monitoring to save their lives. Cardiac arrhythmia classification and prediction have greatly improved in recent years. Arrhythmias are a category of conditions in which the heart's electrical activity is abnormally rapid or sluggish. Every year, it is one of the main reasons of mortality for both men and women, worldwide. For the classification of arrhythmias, this work proposes a novel technique based on optimized feature selection and optimized K-nearest neighbors (KNN) classifier. The proposed method makes advantage of the UCI repository, which has a 279-attribute high-dimensional cardiac arrhythmia dataset. The proposed approach is based on dividing cardiac arrhythmia patients into 16 groups based on the electrocardiography dataset’s features. The purpose is to design an efficient intelligent system employing the dipper throated optimization method to categorize cardiac arrhythmia patients. This method of comprehensive arrhythmia classification outperforms earlier methods presented in the literature. The achieved classification accuracy using the proposed approach is 99.8%.Keywords
Cite This Article
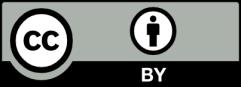
This work is licensed under a Creative Commons Attribution 4.0 International License , which permits unrestricted use, distribution, and reproduction in any medium, provided the original work is properly cited.