Open Access
ARTICLE
Attribute Reduction for Information Systems via Strength of Rules and Similarity Matrix
1 Department of Mathematics, Faculty of Science, Tanta University, Tanta, Egypt
2 Department of Electrical Engineering, Faculty of Engineering, Kafrelsheikh University, Kafrelsheikh, 33516, Egypt
3 Department of Physics and Engineering Mathematics, Faculty of Engineering, Kafrelsheikh University, Kafrelsheikh, 33516, Egypt
* Corresponding Author: Tamer Medhat. Email:
Computer Systems Science and Engineering 2023, 45(2), 1531-1544. https://doi.org/10.32604/csse.2023.031745
Received 26 April 2022; Accepted 08 June 2022; Issue published 03 November 2022
Abstract
An information system is a type of knowledge representation, and attribute reduction is crucial in big data, machine learning, data mining, and intelligent systems. There are several ways for solving attribute reduction problems, but they all require a common categorization. The selection of features in most scientific studies is a challenge for the researcher. When working with huge datasets, selecting all available attributes is not an option because it frequently complicates the study and decreases performance. On the other side, neglecting some attributes might jeopardize data accuracy. In this case, rough set theory provides a useful approach for identifying superfluous attributes that may be ignored without sacrificing any significant information; nonetheless, investigating all available combinations of attributes will result in some problems. Furthermore, because attribute reduction is primarily a mathematical issue, technical progress in reduction is dependent on the advancement of mathematical models. Because the focus of this study is on the mathematical side of attribute reduction, we propose some methods to make a reduction for information systems according to classical rough set theory, the strength of rules and similarity matrix, we applied our proposed methods to several examples and calculate the reduction for each case. These methods expand the options of attribute reductions for researchers.Keywords
Cite This Article
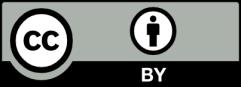
This work is licensed under a Creative Commons Attribution 4.0 International License , which permits unrestricted use, distribution, and reproduction in any medium, provided the original work is properly cited.