Open Access
ARTICLE
Hybrid Metaheuristics Feature Selection with Stacked Deep Learning-Enabled Cyber-Attack Detection Model
1 Department of Computer Science, College of Science & Art at Mahayil, King Khalid University, Muhayel Aseer, 62529, Saudi Arabia
2 Department of Electrical Engineering, College of Engineering, Princess Nourah bint Abdulrahman University, P.O. Box 84428, Riyadh, 11671, Saudi Arabia
3 Department of Computer Sciences, College of Computing and Information System, Umm Al-Qura University, Saudi Arabia
4 Department of Computer Science, College of Sciences and Humanities- Aflaj, Prince Sattam bin Abdulaziz University, Saudi Arabia
5 Department of Digital Media, Faculty of Computers and Information Technology, Future University in Egypt, New Cairo, 11835, Egypt
6 Department of Computer and Self Development, Preparatory Year Deanship, Prince Sattam bin Abdulaziz University, Al-Kharj, 16278, Saudi Arabia
7 Department of Information System, College of Computer Engineering and Sciences, Prince Sattam bin Abdulaziz University, AlKharj, Saudi Arabia
* Corresponding Author: Mesfer Al Duhayyim. Email:
Computer Systems Science and Engineering 2023, 45(2), 1679-1694. https://doi.org/10.32604/csse.2023.031063
Received 09 April 2022; Accepted 08 June 2022; Issue published 03 November 2022
Abstract
Due to exponential increase in smart resource limited devices and high speed communication technologies, Internet of Things (IoT) have received significant attention in different application areas. However, IoT environment is highly susceptible to cyber-attacks because of memory, processing, and communication restrictions. Since traditional models are not adequate for accomplishing security in the IoT environment, the recent developments of deep learning (DL) models find beneficial. This study introduces novel hybrid metaheuristics feature selection with stacked deep learning enabled cyber-attack detection (HMFS-SDLCAD) model. The major intention of the HMFS-SDLCAD model is to recognize the occurrence of cyberattacks in the IoT environment. At the preliminary stage, data pre-processing is carried out to transform the input data into useful format. In addition, salp swarm optimization based on particle swarm optimization (SSOPSO) algorithm is used for feature selection process. Besides, stacked bidirectional gated recurrent unit (SBiGRU) model is utilized for the identification and classification of cyberattacks. Finally, whale optimization algorithm (WOA) is employed for optimal hyperparameter optimization process. The experimental analysis of the HMFS-SDLCAD model is validated using benchmark dataset and the results are assessed under several aspects. The simulation outcomes pointed out the improvements of the HMFS-SDLCAD model over recent approaches.Keywords
Cite This Article
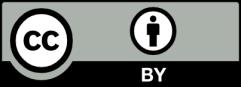
This work is licensed under a Creative Commons Attribution 4.0 International License , which permits unrestricted use, distribution, and reproduction in any medium, provided the original work is properly cited.