Open Access
ARTICLE
Profiling of Urban Noise Using Artificial Intelligence
1 Ph.D. Faculty of Physics, VNU University of Science, Hanoi, 100000, Vietnam
2 University of Science, Vietnam National University, Hanoi, 100000, Vietnam
3 The International School of Penang (Uplands), Penang, Malaysia
4 Alpha School, Hanoi, 100000, Vietnam
* Corresponding Author: Le Quang Thao. Email:
Computer Systems Science and Engineering 2023, 45(2), 1309-1321. https://doi.org/10.32604/csse.2023.031010
Received 08 April 2022; Accepted 26 May 2022; Issue published 03 November 2022
Abstract
Noise pollution tends to receive less awareness compared to other types of pollution, however, it greatly impacts the quality of life for humans such as causing sleep disruption, stress or hearing impairment. Profiling urban sound through the identification of noise sources in cities could help to benefit livability by reducing exposure to noise pollution through methods such as noise control, planning of the soundscape environment, or selection of safe living space. In this paper, we proposed a self-attention long short-term memory (LSTM) method that can improve sound classification compared to previous baselines. An attention mechanism will be designed solely to capture the key section of an audio data series. This is practical as we only need to process important parts of the data and can ignore the rest, making it applicable when gathering information with long-term dependencies. The dataset used is the Urbansound8k dataset which specifically pertains to urban environments and data augmentation was applied to overcome imbalanced data and dataset scarcity. All audio sources in the dataset were normalized to mono signals. From the dataset above, an experiment was conducted to confirm the suitability of the proposed model when applied to the mel-spectrogram and MFCC (Mel-Frequency Cepstral Coefficients) datasets transformed from the original dataset. Improving the classification accuracy depends on the machine learning models as well as the input data, therefore we have evaluated different class models and extraction methods to find the best performing. By combining data augmentation techniques and various extraction methods, our classification model has achieved state-of-the-art performance, each class accuracy is up to 98%.Keywords
Cite This Article
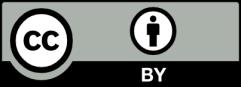