Open Access
ARTICLE
Optimized Deep Learning Model for Effective Spectrum Sensing in Dynamic SNR Scenario
1 Department of Electronics and Communication Engineering, Gnanamani College of Technology, Namakkal, Tamil Nadu, India
2 Department of Electrical and Electronics Engineering, Mahendra Engineering College (Autonomous), Namakkal, Tamil Nadu, India
* Corresponding Author: G. Arunachalam. Email:
Computer Systems Science and Engineering 2023, 45(2), 1279-1294. https://doi.org/10.32604/csse.2023.031001
Received 08 April 2022; Accepted 26 May 2022; Issue published 03 November 2022
Abstract
The main components of Cognitive Radio networks are Primary Users (PU) and Secondary Users (SU). The most essential method used in Cognitive networks is Spectrum Sensing, which detects the spectrum band and opportunistically accesses the free white areas for different users. Exploiting the free spaces helps to increase the spectrum efficiency. But the existing spectrum sensing techniques such as energy detectors, cyclo-stationary detectors suffer from various problems such as complexity, non-responsive behaviors under low Signal to Noise Ratio (SNR) and computational overhead, which affects the performance of the sensing accuracy. Many algorithms such as Long-Short Term Memory (LSTM), Convolutional Neural Networks (CNN), and Recurrent Neural Networks (RNN) play an important role in designing intelligent spectrum sensing techniques due to the excellent learning ability of deep learning frameworks, but still require improvisation in terms of sensing accuracy under dynamic environmental conditions. This paper, we propose the novel and hybrid CNN-Cuttle-Fish Optimized Long Short Term Memory (COLSTM), an improved version of LSTM that is well suited for the dynamic changes of environmental SNR with less computational overhead and complexity. The proposed COLSTM based spectrum sensing technique exploits the various statistical features from spectrum data of PU to improve the sensing efficiency. Furthermore, the addition of shuttle-fish optimization in LSTM has reduced the computational overhead and complexity which in turn enhanced the sensing performances. The proposed methodology is validated on spectrum data acquired using RaspberryPi-RTLSDR experimental test-beds. The proposed spectrum sensing technique and the existing classical spectrum sensing techniques are compared. Experimental results show that the proposed scheme has shown the brighter enhancement of performance under different SNR environments. Further, the improvised performance has been achieved at low complexity and low computational overhead when compared with the other existing LSTM networks.Keywords
Cite This Article
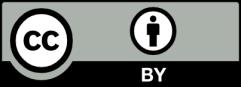