Open Access
ARTICLE
Liver Tumors Segmentation Using 3D SegNet Deep Learning Approach
1 Department of Computer Science and Engineering, PSN College of Engineering and Technology, Tirunelveli, 627152, Tamil Nadu, India
2 Department of Computer Science and Engineering, Gokaraju Rangaraju Institute of Engineering and Technology, Hyderabad, Telangana, 500090, India
3 Department of Computer Science, College of Computers and Information Technology, Taif University, P. O. Box 11099, Taif, 21944, Saudi Arabia
4 Department of Computer Science, College of Computers and Information Technology, Taif University, Taif, 21974, Saudi Arabia
* Corresponding Author: G. Nallasivan. Email:
Computer Systems Science and Engineering 2023, 45(2), 1655-1677. https://doi.org/10.32604/csse.2023.030697
Received 31 March 2022; Accepted 15 June 2022; Issue published 03 November 2022
Abstract
An ultrasonic filter detects signs of malignant tumors by analysing the image’s pixel quality fluctuations caused by a liver ailment. Signs of malignant growth proximity are identified in an ultrasound filter through image pixel quality variations from a liver’s condition. Those changes are more common in alcoholic liver conditions than in other etiologies of cirrhosis, suggesting that the cause may be alcohol instead of liver disease. Existing Two-Dimensional (2D) ultrasound data sets contain an accuracy rate of 85.9% and a 2D Computed Tomography (CT) data set of 91.02%. The most recent work on designing a Three-Dimensional (3D) ultrasound imaging system in or close to real-time is examined. In this article, a Deep Learning (DL) model is implemented and modified to fit liver CT segmentation, and a semantic pixel classification of road scenes is recommended. The architecture is called semantic pixel-wise segmentation and comprises a hierarchical link of encoder-decoder layers. A standard data set was used to test the proposed model for liver CT scans and the tumor accuracy in the training phase. For the normal class, we obtained 100% precision for chronic cirrhosis hepatitis (73%), offset cirrhosis (59.26%), and offensive cirrhosis (91.67%) for chronic hepatitis or cirrhosis (73,0%). The aim is to develop a Computer-Aided Detection (CAD) screening tool to detect steatosis. The results proved 98.33% exactness, 94.59% sensitivity, and 92.11% case with Convolutional Neural Networks (CNN) classification. Although the classifier’s performance did not differentiate so clearly at this level, it was recommended that CNN generally perform better due to the good relationship between Area under the Receiver Operating Characteristics Curve (AUC) and accuracy.Keywords
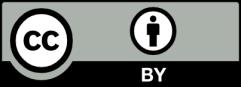