Open Access
ARTICLE
Leveraging Readability and Sentiment in Spam Review Filtering Using Transformer Models
Department of Computer Science and Engineering, National Institute of Technology Puducherry, Karaikal, India
* Corresponding Author: Sujithra Kanmani. Email:
Computer Systems Science and Engineering 2023, 45(2), 1439-1454. https://doi.org/10.32604/csse.2023.029953
Received 15 March 2022; Accepted 30 May 2022; Issue published 03 November 2022
Abstract
Online reviews significantly influence decision-making in many aspects of society. The integrity of internet evaluations is crucial for both consumers and vendors. This concern necessitates the development of effective fake review detection techniques. The goal of this study is to identify fraudulent text reviews. A comparison is made on shill reviews vs. genuine reviews over sentiment and readability features using semi-supervised language processing methods with a labeled and balanced Deceptive Opinion dataset. We analyze textual features accessible in internet reviews by merging sentiment mining approaches with readability. Overall, the research improves fake review screening by using various transformer models such as Bidirectional Encoder Representation from Transformers (BERT), Robustly Optimized BERT (Roberta), XLNET (Transformer-XL) and XLM-Roberta (Cross-lingual Language model–Roberta). This proposed research extracts and classifies features from product reviews to increase the effectiveness of review filtering. As evidenced by the investigation, the application of transformer models improves the performance of spam review filtering when related to existing machine learning and deep learning models.Keywords
Cite This Article
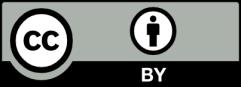