Open Access
ARTICLE
A Machine Learning Approach for Artifact Removal from Brain Signal
Department of Electronics and Communication Engineering, ITER, Sikhsha ‘O’Anusandhan (Deemed to be University), Bhubaneswar, 751030, India
* Corresponding Author: Mihir Narayan Mohanty. Email:
Computer Systems Science and Engineering 2023, 45(2), 1455-1467. https://doi.org/10.32604/csse.2023.029649
Received 08 March 2022; Accepted 27 May 2022; Issue published 03 November 2022
Abstract
Electroencephalography (EEG), helps to analyze the neuronal activity of a human brain in the form of electrical signals with high temporal resolution in the millisecond range. To extract clean clinical information from EEG signals, it is essential to remove unwanted artifacts that are due to different causes including at the time of acquisition. In this piece of work, the authors considered the EEG signal contaminated with Electrocardiogram (ECG) artifacts that occurs mostly in cardiac patients. The clean EEG is taken from the openly available Mendeley database whereas the ECG signal is collected from the Physionet database to create artifacts in the EEG signal and verify the proposed algorithm. Being the artifactual signal is non-linear and non-stationary the Random Vector Functional Link Network (RVFLN) model is used in this case. The Machine Learning approach has taken a leading role in every field of current research and RVFLN is one of them. For the proof of adaptive nature, the model is designed with EEG as a reference and artifactual EEG as input. The peaks of ECG signals are evaluated for artifact estimation as the amplitude is higher than the EEG signal. To vary the weight and reduce the error, an exponentially weighted Recursive Least Square(RLS) algorithm is used to design the adaptive filter with the novel RVFLN model. The random vectors are considered in this model with a radial basis function to satisfy the required signal experimentation. It is found that the result is excellent in terms of Mean Square Error (MSE), Normalized Mean Square Error (NMSE), Relative Error (RE), Gain in Signal to Artifact Ratio (GSAR), Signal Noise Ratio(SNR), Information Quantity (IQ), and Improvement in Normalized Power Spectrum (INPS). Also, the proposed method is compared with the earlier methods to show its efficacy.Keywords
Cite This Article
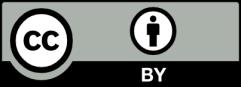