Open Access
ARTICLE
Sea-Land Segmentation of Remote Sensing Images Based on SDW-UNet
1 The Information Department, Beijing University of Technology, Beijing 100124, China
2 School of Physics and Electronic Information Engineering, Qinghai Minzu University, Xining, 810000, China
3 Advanced Information Network Beijing Laboratory, Beijing, 100124, China
4 Computational Intelligence and Intelligent Systems Beijing key Laboratory, Beijing, 100124, China
5 Department of Computer Science, University of Pittsburgh, 15260, USA
* Corresponding Author: Pengyu Liu. Email:
Computer Systems Science and Engineering 2023, 45(2), 1033-1045. https://doi.org/10.32604/csse.2023.028225
Received 05 February 2022; Accepted 07 April 2022; Issue published 03 November 2022
Abstract
Image segmentation of sea-land remote sensing images is of great importance for downstream applications including shoreline extraction, the monitoring of near-shore marine environment, and near-shore target recognition. To mitigate large number of parameters and improve the segmentation accuracy, we propose a new Squeeze-Depth-Wise UNet (SDW-UNet) deep learning model for sea-land remote sensing image segmentation. The proposed SDW-UNet model leverages the squeeze-excitation and depth-wise separable convolution to construct new convolution modules, which enhance the model capacity in combining multiple channels and reduces the model parameters. We further explore the effect of position-encoded information in NLP (Natural Language Processing) domain on sea-land segmentation task. We have conducted extensive experiments to compare the proposed network with the mainstream segmentation network in terms of accuracy, the number of parameters and the time cost for prediction. The test results on remote sensing data sets of Guam, Okinawa, Taiwan, San Diego, and Diego Garcia demonstrate the effectiveness of SDW-UNet in recognizing different types of sea-land areas with a smaller number of parameters, reduces prediction time cost and improves performance over other mainstream segmentation models. We also show that the position encoding can further improve the accuracy of model segmentation.Keywords
Cite This Article
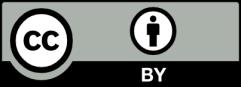
This work is licensed under a Creative Commons Attribution 4.0 International License , which permits unrestricted use, distribution, and reproduction in any medium, provided the original work is properly cited.