Open Access
ARTICLE
Improved Soil Quality Prediction Model Using Deep Learning for Smart Agriculture Systems
1 Department of Information Technology, SNS College of Engineering, Coimbatore, Tamilnadu, India
2 Department of Electrical and Electronics Engineering, SNS College of Technology, Coimbatore, Tamilnadu, India
3 Department of Computer Science & Engineering, SNS College of Technology, Coimbatore, Tamilnadu, India
* Corresponding Author: P. Sumathi. Email:
Computer Systems Science and Engineering 2023, 45(2), 1545-1559. https://doi.org/10.32604/csse.2023.027580
Received 21 January 2022; Accepted 12 May 2022; Issue published 03 November 2022
Abstract
Soil is the major source of infinite lives on Earth and the quality of soil plays significant role on Agriculture practices all around. Hence, the evaluation of soil quality is very important for determining the amount of nutrients that the soil require for proper yield. In present decade, the application of deep learning models in many fields of research has created greater impact. The increasing soil data availability of soil data there is a greater demand for the remotely avail open source model, leads to the incorporation of deep learning method to predict the soil quality. With that concern, this paper proposes a novel model called Improved Soil Quality Prediction Model using Deep Learning (ISQP-DL). The work considers the chemical, physical and biological factors of soil in particular area to estimate the soil quality. Firstly, pH rating of soil samples has been collected from the soil testing laboratory from which the acidic range has been categorized through soil test and the same data has been taken as input to the Deep Neural Network Regression (DNNR) model. Secondly, soil nutrient data has been given as second input to the DNNR model. By utilizing this data set, the DNNR method is used to evaluate the fertility rate by which the soil quality has been estimated. For training and testing, the model uses Deep Neural Network Regression (DNNR), by utilizing the dataset. The results show that the proposed model is effective for SQP (Soil Quality Prediction Model) with efficient good fitting and generality is enhanced with input features with higher rate of classification accuracy. The results show that the proposed model achieves 96.7% of accuracy rate compared with existing models.Keywords
Cite This Article
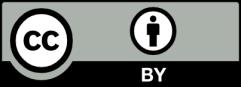
This work is licensed under a Creative Commons Attribution 4.0 International License , which permits unrestricted use, distribution, and reproduction in any medium, provided the original work is properly cited.