Open Access
ARTICLE
Fine Grained Feature Extraction Model of Riot-related Images Based on YOLOv5
1 Department of Information Cyber Security, People’s Public Security University of China, Beijing, 100038, China
2 Key Laboratory of Safety Precautions and Risk Assessment, Ministry of Public Security, Beijing, 102623, China
3 Public Security Behavioral Science Laboratory, People’s Public Security University of China, Beijing, 102623, China
* Corresponding Author: Deyu Yuan. Email:
Computer Systems Science and Engineering 2023, 45(1), 85-97. https://doi.org/10.32604/csse.2023.030849
Received 03 April 2022; Accepted 26 May 2022; Issue published 16 August 2022
Abstract
With the rapid development of Internet technology, the type of information in the Internet is extremely complex, and a large number of riot contents containing bloody, violent and riotous components have appeared. These contents pose a great threat to the network ecology and national security. As a result, the importance of monitoring riotous Internet activity cannot be overstated. Convolutional Neural Network (CNN-based) target detection algorithm has great potential in identifying rioters, so this paper focused on the use of improved backbone and optimization function of You Only Look Once v5 (YOLOv5), and further optimization of hyperparameters using genetic algorithm to achieve fine-grained recognition of riot image content. First, the fine-grained features of riot-related images were identified, and then the dataset was constructed by manual annotation. Second, the training and testing work was carried out on the constructed dedicated dataset by supervised deep learning training. The research results have shown that the improved YOLOv5 network significantly improved the fine-grained feature extraction capability of riot-related images compared with the original YOLOv5 network structure, and the mean average precision (mAP) value was improved to 0.6128. Thus, it provided strong support for combating riot-related organizations and maintaining the online ecological environment.Keywords
Cite This Article
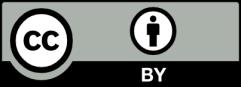
This work is licensed under a Creative Commons Attribution 4.0 International License , which permits unrestricted use, distribution, and reproduction in any medium, provided the original work is properly cited.