Open Access
ARTICLE
Hybrid Bacterial Foraging Optimization with Sparse Autoencoder for Energy Systems
1 Business Analytics, School of Business and Management, Christ University, Bangalore, 560029, India
2 Department of Computer Science and IT, School of Sciences, Jain Deemed to be University, Bangalore, 560027, India
3 Department of Computer Science and Engineering, Karunya Institute of Technology and Sciences, Coimbatore, 641114, India
4 College of Computer Studies, University of Technology Bahrain, 18041, Bahrain
5 Institute of Computer Science and Engineering, Saveetha School of Engineering, Saveetha Institute of Medical and Technical Sciences, Chennai, 602105, India
6 Department of Computer Science and Engineering, Panimalar Institute of Technology, Chennai, 600123, India
7 Department of Information Science & Engineering, CMR Institute of Technology, Bengaluru, 560037, India
* Corresponding Author: Joshua Samuel Raj. Email:
Computer Systems Science and Engineering 2023, 45(1), 701-714. https://doi.org/10.32604/csse.2023.030611
Received 29 March 2022; Accepted 04 May 2022; Issue published 16 August 2022
Abstract
The Internet of Things (IoT) technologies has gained significant interest in the design of smart grids (SGs). The increasing amount of distributed generations, maturity of existing grid infrastructures, and demand network transformation have received maximum attention. An essential energy storing model mostly the electrical energy stored methods are developing as the diagnoses for its procedure was becoming further compelling. The dynamic electrical energy stored model using Electric Vehicles (EVs) is comparatively standard because of its excellent electrical property and flexibility however the chance of damage to its battery was there in event of overcharging or deep discharging and its mass penetration deeply influences the grids. This paper offers a new Hybridization of Bacterial foraging optimization with Sparse Autoencoder (HBFOA-SAE) model for IoT Enabled energy systems. The proposed HBFOA-SAE model majorly intends to effectually estimate the state of charge (SOC) values in the IoT based energy system. To accomplish this, the SAE technique was executed to proper determination of the SOC values in the energy systems. Next, for improving the performance of the SOC estimation process, the HBFOA is employed. In addition, the HBFOA technique is derived by the integration of the hill climbing (HC) concepts with the BFOA to improve the overall efficiency. For ensuring better outcomes for the HBFOA-SAE model, a comprehensive set of simulations were performed and the outcomes are inspected under several aspects. The experimental results reported the supremacy of the HBFOA-SAE model over the recent state of art approaches.Keywords
Cite This Article
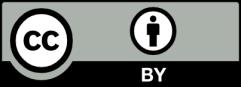
This work is licensed under a Creative Commons Attribution 4.0 International License , which permits unrestricted use, distribution, and reproduction in any medium, provided the original work is properly cited.