Open Access
ARTICLE
Classification Model for IDS Using Auto Cryptographic Denoising Technique
1 Department of Computer Science & Engineering, PPG Institute of Technology, Coimbatore, Tamilnadu, India
2 Department of Computer Science & Engineering, SNS College of Technology, Coimbatore, Tamilnadu, India
* Corresponding Author: P. Sivaprakash. Email:
Computer Systems Science and Engineering 2023, 45(1), 671-685. https://doi.org/10.32604/csse.2023.029984
Received 16 March 2022; Accepted 28 April 2022; Issue published 16 August 2022
Abstract
Intrusion detection systems (IDS) are one of the most promising ways for securing data and networks; In recent decades, IDS has used a variety of categorization algorithms. These classifiers, on the other hand, do not work effectively unless they are combined with additional algorithms that can alter the classifier’s parameters or select the optimal sub-set of features for the problem. Optimizers are used in tandem with classifiers to increase the stability and with efficiency of the classifiers in detecting invasion. These algorithms, on the other hand, have a number of limitations, particularly when used to detect new types of threats. In this paper, the NSL KDD dataset and KDD Cup 99 is used to find the performance of the proposed classifier model and compared; These two IDS dataset is preprocessed, then Auto Cryptographic Denoising (ACD) adopted to remove noise in the feature of the IDS dataset; the classifier algorithms, K-Means and Neural network classifies the dataset with adam optimizer. IDS classifier is evaluated by measuring performance measures like f-measure, recall, precision, detection rate and accuracy. The neural network obtained the highest classifying accuracy as 91.12% with drop-out function that shows the efficiency of the classifier model with drop-out function for KDD Cup99 dataset. Explaining their power and limitations in the proposed methodology that could be used in future works in the IDS area.Keywords
Cite This Article
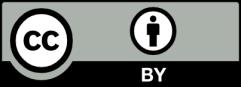