Open Access
ARTICLE
Early Detection of Heartbeat from Multimodal Data Using RPA Learning with KDNN-SAE
1 Department of Electronics and Communication Engineering, Ponjesly College of Engineering, Nagercoil, India
2 Department of Electronics and Communication Engineering, CSI Institute of Technology, Nagercoil, India
* Corresponding Author: A. K. S. Saranya. Email:
Computer Systems Science and Engineering 2023, 45(1), 545-562. https://doi.org/10.32604/csse.2023.029975
Received 16 March 2022; Accepted 20 April 2022; Issue published 16 August 2022
Abstract
Heartbeat detection stays central to cardiovascular an electrocardiogram (ECG) is used to help with disease diagnosis and management. Existing Convolutional Neural Network (CNN)-based methods suffer from the less generalization problem thus; the effectiveness and robustness of the traditional heartbeat detector methods cannot be guaranteed. In contrast, this work proposes a heartbeat detector Krill based Deep Neural Network Stacked Auto Encoders (KDNN-SAE) that computes the disease before the exact heart rate by combining features from multiple ECG Signals. Heartbeats are classified independently and multiple signals are fused to estimate life threatening conditions earlier without any error in classification of heart beat. This work contained Training and testing stages, in the preparation part at first the Adaptive Filter Enthalpy-based Empirical Mode Decomposition (EMD) is utilized to eliminate the motion artifact in the signal. At that point, the robotic process automation (RPA) learning part extracts the effective features are extracted, and normalized the value of the feature then estimated utilizing the RPA loss function. At last KDNN-SAE prepared training for the data stored in the dataset. In the subsequent stage, input signal compute motion artifact and RPA Learning the evaluation part determines the detection of Heartbeat. So early diagnosis of heart failures is an essential factor. The results of the experiments show that our proposed method has a high score outcome of 0.9997. Comparable to the CIF, which reaches 0.9990. The CNN and Artificial Neural Network (ANN) had less score 0.95115 and 0.90147.Keywords
Cite This Article
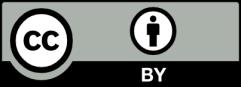