Open Access
ARTICLE
Machine Learning-based Inverse Model for Few-Mode Fiber Designs
1 ITER, Siksha ‘O’ Anusandhan (Deemed to be University), Bhubaneswar, 751030, India
2 Department of Electronics and Electrical Communication Engineering, IIT, Kharagpur, 721302, India
* Corresponding Author: Mihir Narayan Mohanty. Email:
Computer Systems Science and Engineering 2023, 45(1), 311-328. https://doi.org/10.32604/csse.2023.029325
Received 01 March 2022; Accepted 12 April 2022; Issue published 16 August 2022
Abstract
The medium for next-generation communication is considered as fiber for fast, secure communication and switching capability. Mode division and space division multiplexing provide an excellent switching capability with high data transmission rate. In this work, the authors have approached an inverse modeling technique using regression-based machine learning to design a weakly coupled few-mode fiber for facilitating mode division multiplexing. The technique is adapted to predict the accurate profile parameters for the proposed few-mode fiber to obtain the maximum number of modes. It is for a three-ring-core few-mode fiber for guiding five, ten, fifteen, and twenty modes. Three types of regression models namely ordinary least-square linear multi-output regression, k-nearest neighbors of multi-output regression, and ID3 algorithm-based decision trees for multi-output regression are used for predicting the multiple profile parameters. It is observed that the ID3-based decision tree for multioutput regression is the robust, highly-accurate machine learning model for fast modeling of FMFs. The proposed fiber claims to be an efficient candidate for the next-generation 5G and 6G backhaul networks using mode division multiplexing.Keywords
Cite This Article
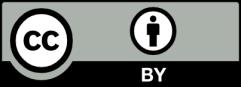
This work is licensed under a Creative Commons Attribution 4.0 International License , which permits unrestricted use, distribution, and reproduction in any medium, provided the original work is properly cited.