Open Access
ARTICLE
Q-Learning-Based Pesticide Contamination Prediction in Vegetables and Fruits
Department of Computer Science and Engineering, KPR Institute of Engineering and Technology, Coimbatore, 641407, Tamil Nadu, India
* Corresponding Author: Kandasamy Sellamuthu. Email:
Computer Systems Science and Engineering 2023, 45(1), 715-736. https://doi.org/10.32604/csse.2023.029017
Received 22 February 2022; Accepted 13 April 2022; Issue published 16 August 2022
Abstract
Pesticides have become more necessary in modern agricultural production. However, these pesticides have an unforeseeable long-term impact on people's wellbeing as well as the ecosystem. Due to a shortage of basic pesticide exposure awareness, farmers typically utilize pesticides extremely close to harvesting. Pesticide residues within foods, particularly fruits as well as veggies, are a significant issue among farmers, merchants, and particularly consumers. The residual concentrations were far lower than these maximal allowable limits, with only a few surpassing the restrictions for such pesticides in food. There is an obligation to provide a warning about this amount of pesticide use in farming. Previous technologies failed to forecast the large number of pesticides that were dangerous to people, necessitating the development of improved detection and early warning systems. A novel methodology for verifying the status and evaluating the level of pesticides in regularly consumed veggies as well as fruits has been identified, named as the Hybrid Chronic Multi-Residual Framework (HCMF), in which the harmful level of used pesticide residues has been predicted for contamination in agro products using Q-Learning based Recurrent Neural Network and the predicted contamination levels have been analyzed using Complex Event Processing (CEP) by processing given spatial and sequential data. The analysis results are used to minimize and effectively use pesticides in the agricultural field and also ensure the safety of farmers and consumers. Overall, the technique is carried out in a Python environment, with the results showing that the proposed model has a 98.57% accuracy and a training loss of 0.30.Keywords
Cite This Article
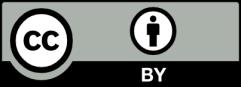
This work is licensed under a Creative Commons Attribution 4.0 International License , which permits unrestricted use, distribution, and reproduction in any medium, provided the original work is properly cited.