Open Access
ARTICLE
Super-Resolution Based on Curvelet Transform and Sparse Representation
1 Department of Computer Science, Faculty of Computers and Informatics, Suez Canal University, Ismailia, 51422, Egypt
2 Department of Information Technology, University of Jeddah, College of Computing and Information Technology at Khulais, Jeddah, Saudi Arabia
* Corresponding Author: Israa Ismail. Email:
Computer Systems Science and Engineering 2023, 45(1), 167-181. https://doi.org/10.32604/csse.2023.028906
Received 21 February 2022; Accepted 06 April 2022; Issue published 16 August 2022
Abstract
Super-resolution techniques are used to reconstruct an image with a high resolution from one or more low-resolution image(s). In this paper, we proposed a single image super-resolution algorithm. It uses the nonlocal mean filter as a prior step to produce a denoised image. The proposed algorithm is based on curvelet transform. It converts the denoised image into low and high frequencies (sub-bands). Then we applied a multi-dimensional interpolation called Lancozos interpolation over both sub-bands. In parallel, we applied sparse representation with over complete dictionary for the denoised image. The proposed algorithm then combines the dictionary learning in the sparse representation and the interpolated sub-bands using inverse curvelet transform to have an image with a higher resolution. The experimental results of the proposed super-resolution algorithm show superior performance and obviously better-recovering images with enhanced edges. The comparison study shows that the proposed super-resolution algorithm outperforms the state-of-the-art. The mean absolute error is 0.021 ± 0.008 and the structural similarity index measure is 0.89 ± 0.08.Keywords
Cite This Article
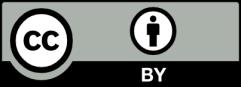
This work is licensed under a Creative Commons Attribution 4.0 International License , which permits unrestricted use, distribution, and reproduction in any medium, provided the original work is properly cited.