Open Access
ARTICLE
Detection of Diabetic Retinopathy from Retinal Images Using DenseNet Models
1 Department of ECE, K S R Institute for Engineering and Technology, Tiruchengode, 637215, India
2 Department of Computer Science and Engineering, SRM Institute of Science and Technology, Chennai, 603203, India
3 Department of Electronics and Communication and Engineering, SRM Institute of Science and Technology, Chennai, 603203, India
* Corresponding Author: P. Saranya. Email:
Computer Systems Science and Engineering 2023, 45(1), 279-292. https://doi.org/10.32604/csse.2023.028703
Received 16 February 2022; Accepted 18 April 2022; Issue published 16 August 2022
Abstract
A prevalent diabetic complication is Diabetic Retinopathy (DR), which can damage the retina’s veins, leading to a severe loss of vision. If treated in the early stage, it can help to prevent vision loss. But since its diagnosis takes time and there is a shortage of ophthalmologists, patients suffer vision loss even before diagnosis. Hence, early detection of DR is the necessity of the time. The primary purpose of the work is to apply the data fusion/feature fusion technique, which combines more than one relevant feature to predict diabetic retinopathy at an early stage with greater accuracy. Mechanized procedures for diabetic retinopathy analysis are fundamental in taking care of these issues. While profound learning for parallel characterization has accomplished high approval exactness’s, multi-stage order results are less noteworthy, especially during beginning phase sickness. Densely Connected Convolutional Networks are suggested to detect of Diabetic Retinopathy on retinal images. The presented model is trained on a Diabetic Retinopathy Dataset having 3,662 images given by APTOS. Experimental results suggest that the training accuracy of 93.51% 0.98 precision, 0.98 recall and 0.98 F1-score has been achieved through the best one out of the three models in the proposed work. The same model is tested on 550 images of the Kaggle 2015 dataset where the proposed model was able to detect No DR images with 96% accuracy, Mild DR images with 90% accuracy, Moderate DR images with 89% accuracy, Severe DR images with 87% accuracy and Proliferative DR images with 93% accuracy.Keywords
Cite This Article
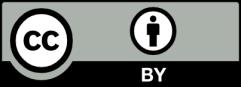