Open Access
ARTICLE
A Double Threshold Energy Detection-Based Neural Network for Cognitive Radio Networks
1 Department of Electrical and Electronics Engineering, Red Sea University, Port Sudan, 33311, Sudan
2 Department of Information Technology, College of Computer and Information Sciences, Princess Nourah bint Abdulrahman University, Riyadh, 11671, Saudi Arabia
3 Department of Information Technology, College of Computing and Informatics, Saudi Electronic University, Riyadh, 93499, Saudi Arabia
4 Department of Computer Engineering, College of Computers and Information Technology, Taif University, Taif, 21944, Saudi Arabia
5 Department of Electronics Engineering, Sudan University of Science and Technology, Khartoum, 11111, Sudan
* Corresponding Author: Raed Alsaqour. Email:
Computer Systems Science and Engineering 2023, 45(1), 329-342. https://doi.org/10.32604/csse.2023.028528
Received 11 February 2022; Accepted 10 April 2022; Issue published 16 August 2022
Abstract
In cognitive radio networks (CoR), the performance of cooperative spectrum sensing is improved by reducing the overall error rate or maximizing the detection probability. Several optimization methods are usually used to optimize the number of user-chosen for cooperation and the threshold selection. However, these methods do not take into account the effect of sample size and its effect on improving CoR performance. In general, a large sample size results in more reliable detection, but takes longer sensing time and increases complexity. Thus, the locally sensed sample size is an optimization problem. Therefore, optimizing the local sample size for each cognitive user helps to improve CoR performance. In this study, two new methods are proposed to find the optimum sample size to achieve objective-based improved (single/double) threshold energy detection, these methods are the optimum sample size N* and neural networks (NN) optimization. Through the evaluation, it was found that the proposed methods outperform the traditional sample size selection in terms of the total error rate, detection probability, and throughput.
Keywords
Cite This Article
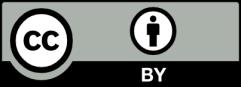