Open Access
ARTICLE
Hybrid Deep Learning-Improved BAT Optimization Algorithm for Soil Classification Using Hyperspectral Features
1 Saveetha School of Engineering, Saveetha Institute of Medical and Technical Sciences, Saveetha Nagar, Thandalam, Chennai, 602105, Tamilnadu, India
2 Department of Electronics and Communication Engineering, College of Engineering & Technology, SRM Institute of Science and Technology, Vadapalani Campus, Chennai, 600026, India
* Corresponding Author: S. Srinivasan. Email:
Computer Systems Science and Engineering 2023, 45(1), 579-594. https://doi.org/10.32604/csse.2023.027592
Received 21 January 2022; Accepted 02 March 2022; Issue published 16 August 2022
Abstract
Now a days, Remote Sensing (RS) techniques are used for earth observation and for detection of soil types with high accuracy and better reliability. This technique provides perspective view of spatial resolution and aids in instantaneous measurement of soil’s minerals and its characteristics. There are a few challenges that is present in soil classification using image enhancement such as, locating and plotting soil boundaries, slopes, hazardous areas, drainage condition, land use, vegetation etc. There are some traditional approaches which involves few drawbacks such as, manual involvement which results in inaccuracy due to human interference, time consuming, inconsistent prediction etc. To overcome these draw backs and to improve the predictive analysis of soil characteristics, we propose a Hybrid Deep Learning improved BAT optimization algorithm (HDIB) for soil classification using remote sensing hyperspectral features. In HDIB, we propose a spontaneous BAT optimization algorithm for feature extraction of both spectral-spatial features by choosing pure pixels from the Hyper Spectral (HS) image. Spectral-spatial vector as training illustrations is attained by merging spatial and spectral vector by means of priority stacking methodology. Then, a recurring Deep Learning (DL) Neural Network (NN) is used for classifying the HS images, considering the datasets of Pavia University, Salinas and Tamil Nadu Hill Scene, which in turn improves the reliability of classification. Finally, the performance of the proposed HDIB based soil classifier is compared and analyzed with existing methodologies like Single Layer Perceptron (SLP), Convolutional Neural Networks (CNN) and Deep Metric Learning (DML) and it shows an improved classification accuracy of 99.87%, 98.34% and 99.9% for Tamil Nadu Hills dataset, Pavia University and Salinas scene datasets respectively.Keywords
Cite This Article
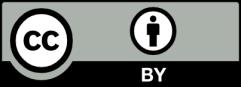
This work is licensed under a Creative Commons Attribution 4.0 International License , which permits unrestricted use, distribution, and reproduction in any medium, provided the original work is properly cited.