Open Access
ARTICLE
Ext-ICAS: A Novel Self-Normalized Extractive Intra Cosine Attention Similarity Summarization
1 Deparment of Information Technology, Thiagarajar College of Engineering, Madurai, India
2 Deparment of Data Science, Thiagarajar College of Engineering, Madurai, India
* Corresponding Author: P. Sharmila. Email:
Computer Systems Science and Engineering 2023, 45(1), 377-393. https://doi.org/10.32604/csse.2023.027481
Received 18 January 2022; Accepted 08 April 2022; Issue published 16 August 2022
Abstract
With the continuous growth of online news articles, there arises the necessity for an efficient abstractive summarization technique for the problem of information overloading. Abstractive summarization is highly complex and requires a deeper understanding and proper reasoning to come up with its own summary outline. Abstractive summarization task is framed as seq2seq modeling. Existing seq2seq methods perform better on short sequences; however, for long sequences, the performance degrades due to high computation and hence a two-phase self-normalized deep neural document summarization model consisting of improvised extractive cosine normalization and seq2seq abstractive phases has been proposed in this paper. The novelty is to parallelize the sequence computation training by incorporating feed-forward, the self-normalized neural network in the Extractive phase using Intra Cosine Attention Similarity (Ext-ICAS) with sentence dependency position. Also, it does not require any normalization technique explicitly. Our proposed abstractive Bidirectional Long Short Term Memory (Bi-LSTM) encoder sequence model performs better than the Bidirectional Gated Recurrent Unit (Bi-GRU) encoder with minimum training loss and with fast convergence. The proposed model was evaluated on the Cable News Network (CNN)/Daily Mail dataset and an average rouge score of 0.435 was achieved also computational training in the extractive phase was reduced by 59% with an average number of similarity computations.Keywords
Cite This Article
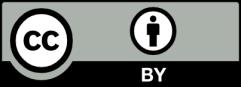
This work is licensed under a Creative Commons Attribution 4.0 International License , which permits unrestricted use, distribution, and reproduction in any medium, provided the original work is properly cited.