Open Access
ARTICLE
An Efficient Unsupervised Learning Approach for Detecting Anomaly in Cloud
1 Department of Information Technology, Karpagam College of Engineering, Coimbatore, Tamilnadu, India
2 Department of Computer Science and Engineering, Karpagam Academy of Higher Education, Coimbatore, Tamilnadu, India
3 Department of Computer Science and Engineering, Koneru Lakshmaiah Education Foundation, Andhra Pradesh, India
4 Department of MCA, CMR Institute of Technology, Bengaluru, Karnataka, India
5 Department of Computer Science and Engineering, Prasad V. Potluri Siddhartha Institute of Technology, Andhra Pradesh, India
6 Department of Computer Science and Engineering, Residency College, Chennai, India
* Corresponding Author: P. Sherubha. Email:
Computer Systems Science and Engineering 2023, 45(1), 149-166. https://doi.org/10.32604/csse.2023.024424
Received 16 October 2021; Accepted 30 December 2021; Issue published 16 August 2022
Abstract
The Cloud system shows its growing functionalities in various industrial applications. The safety towards data transfer seems to be a threat where Network Intrusion Detection System (NIDS) is measured as an essential element to fulfill security. Recently, Machine Learning (ML) approaches have been used for the construction of intellectual IDS. Most IDS are based on ML techniques either as unsupervised or supervised. In supervised learning, NIDS is based on labeled data where it reduces the efficiency of the reduced model to identify attack patterns. Similarly, the unsupervised model fails to provide a satisfactory outcome. Hence, to boost the functionality of unsupervised learning, an effectual auto-encoder is applied for feature selection to select good features. Finally, the Naïve Bayes classifier is used for classification purposes. This approach exposes the finest generalization ability to train the data. The unlabelled data is also used for adoption towards data analysis. Here, redundant and noisy samples over the dataset are eliminated. To validate the robustness and efficiency of NIDS, the anticipated model is tested over the NSL-KDD dataset. The experimental outcomes demonstrate that the anticipated approach attains superior accuracy with 93%, which is higher compared to J48, AB tree, Random Forest (RF), Regression Tree (RT), Multi-Layer Perceptrons (MLP), Support Vector Machine (SVM), and Fuzzy. Similarly, False Alarm Rate (FAR) and True Positive Rate (TPR) of Naive Bayes (NB) is 0.3 and 0.99, respectively. When compared to prevailing techniques, the anticipated approach also delivers promising outcomes.Keywords
Cite This Article
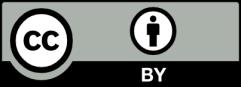