Open Access
ARTICLE
Deep Learning with Natural Language Processing Enabled Sentimental Analysis on Sarcasm Classification
1 Department of Documents and Archive, Center of Documents and Administrative Communication, King Faisal University, Al Hofuf, Al-Ahsa, 31982, Saudi Arabia
2 School of Electrical and Electronic Engineering, Engineering Campus, Universiti Sains Malaysia (USM), Nibong Tebal, Penang, 14300, Malaysia
* Corresponding Author: Abdul Rahaman Wahab Sait. Email:
Computer Systems Science and Engineering 2023, 44(3), 2553-2567. https://doi.org/10.32604/csse.2023.029603
Received 07 March 2022; Accepted 07 April 2022; Issue published 01 August 2022
Abstract
Sentiment analysis (SA) is the procedure of recognizing the emotions related to the data that exist in social networking. The existence of sarcasm in textual data is a major challenge in the efficiency of the SA. Earlier works on sarcasm detection on text utilize lexical as well as pragmatic cues namely interjection, punctuations, and sentiment shift that are vital indicators of sarcasm. With the advent of deep-learning, recent works, leveraging neural networks in learning lexical and contextual features, removing the need for handcrafted feature. In this aspect, this study designs a deep learning with natural language processing enabled SA (DLNLP-SA) technique for sarcasm classification. The proposed DLNLP-SA technique aims to detect and classify the occurrence of sarcasm in the input data. Besides, the DLNLP-SA technique holds various sub-processes namely preprocessing, feature vector conversion, and classification. Initially, the pre-processing is performed in diverse ways such as single character removal, multi-spaces removal, URL removal, stopword removal, and tokenization. Secondly, the transformation of feature vectors takes place using the N-gram feature vector technique. Finally, mayfly optimization (MFO) with multi-head self-attention based gated recurrent unit (MHSA-GRU) model is employed for the detection and classification of sarcasm. To verify the enhanced outcomes of the DLNLP-SA model, a comprehensive experimental investigation is performed on the News Headlines Dataset from Kaggle Repository and the results signified the supremacy over the existing approaches.Keywords
Cite This Article
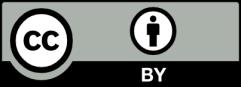
This work is licensed under a Creative Commons Attribution 4.0 International License , which permits unrestricted use, distribution, and reproduction in any medium, provided the original work is properly cited.