Open Access
ARTICLE
Optimal Machine Learning Enabled Performance Monitoring for Learning Management Systems
1 Department of Computer Science and Information Systems, College of Applied Sciences, AlMaarefa University, Ad Diriyah, 13713, Riyadh, Kingdom of Saudi Arabia
2 Physical Therapy Department, Majmaah University, Majmaah, 11952, Kingdom of Saudi Arabia
3 Department of Computer Engineering, College of Computers and Information Technology, Taif University, Taif, 21944, Kingdom of Saudi Arabia
4 Department of Archives and Communication, King Faisal University, Al Ahsa, 31982, Hofuf, Kingdom of Saudi Arabia
5 Department of Computing, Arabeast Colleges, Riyadh, 11583, Kingdom of Saudi Arabia
* Corresponding Author: Ashit Kumar Dutta. Email:
Computer Systems Science and Engineering 2023, 44(3), 2277-2292. https://doi.org/10.32604/csse.2023.028107
Received 02 February 2022; Accepted 30 March 2022; Issue published 01 August 2022
Abstract
Learning Management System (LMS) is an application software that is used in automation, delivery, administration, tracking, and reporting of courses and programs in educational sector. The LMS which exploits machine learning (ML) has the ability of accessing user data and exploit it for improving the learning experience. The recently developed artificial intelligence (AI) and ML models helps to accomplish effective performance monitoring for LMS. Among the different processes involved in ML based LMS, feature selection and classification processes find beneficial. In this motivation, this study introduces Glowworm-based Feature Selection with Machine Learning Enabled Performance Monitoring (GSO-MFWELM) technique for LMS. The key objective of the proposed GSO-MFWELM technique is to effectually monitor the performance in LMS. The proposed GSO-MFWELM technique involves GSO-based feature selection technique to select the optimal features. Besides, Weighted Extreme Learning Machine (WELM) model is applied for classification process whereas the parameters involved in WELM model are optimally fine-tuned with the help of Mayfly Optimization (MFO) algorithm. The design of GSO and MFO techniques result in reduced computation complexity and improved classification performance. The presented GSO-MFWELM technique was validated for its performance against benchmark dataset and the results were inspected under several aspects. The simulation results established the supremacy of GSO-MFWELM technique over recent approaches with the maximum classification accuracy of 0.9589.Keywords
Cite This Article
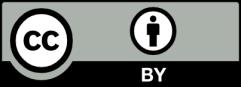
This work is licensed under a Creative Commons Attribution 4.0 International License , which permits unrestricted use, distribution, and reproduction in any medium, provided the original work is properly cited.