Open Access
ARTICLE
Colliding Bodies Optimization with Machine Learning Based Parkinson’s Disease Diagnosis
1 Department of Computer Science and Information Systems, College of Applied Sciences, AlMaarefa University, Ad Diriyah, Riyadh, 13713, Kingdom of Saudi Arabia
2 Department of Nursing, College of Applied Sciences, AlMaarefa University, Ad Diriyah, Riyadh, 13713, Kingdom of Saudi Arabia
3 Department of Computer Engineering, College of Computers and Information Technology, Taif University, Taif, 21944, Kingdom of Saudi Arabia
4 Department of Archives and Communication, King Faisal University, Al Ahsa, Hofuf, 31982, Kingdom of Saudi Arabia
* Corresponding Author: Ashit Kumar Dutta. Email:
Computer Systems Science and Engineering 2023, 44(3), 2195-2207. https://doi.org/10.32604/csse.2023.026461
Received 27 December 2021; Accepted 30 March 2022; Issue published 01 August 2022
Abstract
Parkinson’s disease (PD) is one of the primary vital degenerative diseases that affect the Central Nervous System among elderly patients. It affect their quality of life drastically and millions of seniors are diagnosed with PD every year worldwide. Several models have been presented earlier to detect the PD using various types of measurement data like speech, gait patterns, etc. Early identification of PD is important owing to the fact that the patient can offer important details which helps in slowing down the progress of PD. The recently-emerging Deep Learning (DL) models can leverage the past data to detect and classify PD. With this motivation, the current study develops a novel Colliding Bodies Optimization Algorithm with Optimal Kernel Extreme Learning Machine (CBO-OKELM) for diagnosis and classification of PD. The goal of the proposed CBO-OKELM technique is to identify whether PD exists or not. CBO-OKELM technique involves the design of Colliding Bodies Optimization-based Feature Selection (CBO-FS) technique for optimal subset of features. In addition, Water Strider Algorithm (WSA) with Kernel Extreme Learning Machine (KELM) model is also developed for the classification of PD. CBO algorithm is used to elect the optimal set of features whereas WSA is utilized for parameter tuning of KELM model which altogether helps in accomplishing the maximum PD diagnostic performance. The experimental analysis was conducted for CBO-OKELM technique against four benchmark datasets and the model portrayed better performance such as 95.68%, 96.34%, 92.49%, and 92.36% on Speech PD, Voice PD, Hand PD Meander, and Hand PD Spiral datasets respectively.Keywords
Cite This Article
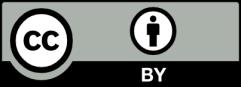
This work is licensed under a Creative Commons Attribution 4.0 International License , which permits unrestricted use, distribution, and reproduction in any medium, provided the original work is properly cited.