Open Access
ARTICLE
An Ordinal Multi-Dimensional Classification (OMDC) for Predictive Maintenance
Izmir Bakircay University, Department of Computer Engineering, Izmir, 35665, Turkey
* Corresponding Author: Pelin Yildirim Taser. Email:
Computer Systems Science and Engineering 2023, 44(2), 1499-1516. https://doi.org/10.32604/csse.2023.028083
Received 02 February 2022; Accepted 04 March 2022; Issue published 15 June 2022
Abstract
Predictive Maintenance is a type of condition-based maintenance that assesses the equipment's states and estimates its failure probability and when maintenance should be performed. Although machine learning techniques have been frequently implemented in this area, the existing studies disregard to the natural order between the target attribute values of the historical sensor data. Thus, these methods cause losing the inherent order of the data that positively affects the prediction performances. To deal with this problem, a novel approach, named Ordinal Multi-dimensional Classification (OMDC), is proposed for estimating the conditions of a hydraulic system's four components by taking into the natural order of class values. To demonstrate the prediction ability of the proposed approach, eleven different multi-dimensional classification algorithms (traditional Binary Relevance (BR), Classifier Chain (CC), Bayesian Classifier Chain (BCC), Monte Carlo Classifier Chain (MCC), Probabilistic Classifier Chain (PCC), Classifier Dependency Network (CDN), Classifier Trellis (CT), Classifier Dependency Trellis (CDT), Label Powerset (LP), Pruned Sets (PS), and Random k-Labelsets (RAKEL)) were implemented using the Ordinal Class Classifier (OCC) algorithm. Besides, seven different classification algorithms (Multilayer Perceptron (MLP), Support Vector Machine (SVM), k-Nearest Neighbour (kNN), Decision Tree (C4.5), Bagging, Random Forest (RF), and Adaptive Boosting (AdaBoost)) were chosen as base learners for the OCC algorithm. The experimental results present that the proposed OMDC approach using binary relevance multi-dimensional classification methods predicts the conditions of a hydraulic system's multiple components with high accuracy. Also, it is clearly seen from the results that the OMDC models that utilize ensemble-based classification algorithms give more reliable prediction performances with an average Hamming score of 0.853 than the others that use traditional algorithms as base learners.Keywords
Cite This Article
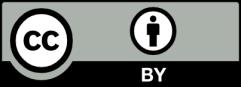