Open Access
ARTICLE
An Optimized Transfer Learning Model Based Kidney Stone Classification
Department of Computer Science and Engineering, Mepco Schlenk Engineering College, Sivakasi, 626005, Tamilnadu, India
* Corresponding Author: S. Devi Mahalakshmi. Email:
Computer Systems Science and Engineering 2023, 44(2), 1387-1395. https://doi.org/10.32604/csse.2023.027610
Received 21 January 2022; Accepted 23 February 2022; Issue published 15 June 2022
Abstract
The kidney is an important organ of humans to purify the blood. The healthy function of the kidney is always essential to balance the salt, potassium and pH levels in the blood. Recently, the failure of kidneys happens easily to human beings due to their lifestyle, eating habits and diabetes diseases. Early prediction of kidney stones is compulsory for timely treatment. Image processing-based diagnosis approaches provide a greater success rate than other detection approaches. In this work, proposed a kidney stone classification method based on optimized Transfer Learning(TL). The Deep Convolutional Neural Network (DCNN) models of DenseNet169, MobileNetv2 and GoogleNet applied for classification. The combined classification results are processed by ensemble learning to increase classification performance. The hyperparameters of the DCNN model are adjusted by the metaheuristic algorithm of Gorilla Troops Optimizer (GTO). The proposed TL model outperforms in terms of all the parameters compared to other DCNN models.Keywords
Cite This Article
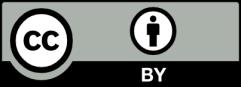