Open Access
ARTICLE
Efficient Object Detection and Classification Approach Using HTYOLOV4 and M2RFO-CNN
Department of Information Technology, Anna University, MIT Campus, Chennai, 600044, India
* Corresponding Author: V. Arulalan. Email:
Computer Systems Science and Engineering 2023, 44(2), 1703-1717. https://doi.org/10.32604/csse.2023.026744
Received 04 January 2022; Accepted 10 March 2022; Issue published 15 June 2022
Abstract
Object detection and classification are the trending research topics in the field of computer vision because of their applications like visual surveillance. However, the vision-based objects detection and classification methods still suffer from detecting smaller objects and dense objects in the complex dynamic environment with high accuracy and precision. The present paper proposes a novel enhanced method to detect and classify objects using Hyperbolic Tangent based You Only Look Once V4 with a Modified Manta-Ray Foraging Optimization-based Convolution Neural Network. Initially, in the pre-processing, the video data was converted into image sequences and Polynomial Adaptive Edge was applied to preserve the Algorithm method for image resizing and noise removal. The noiseless resized image sequences contrast was enhanced using Contrast Limited Adaptive Edge Preserving Algorithm. And, with the contrast-enhanced image sequences, the Hyperbolic Tangent based You Only Look Once V4 was trained for object detection. Additionally, to detect smaller objects with high accuracy, Grasp configuration was observed for every detected object. Finally, the Modified Manta-Ray Foraging Optimization-based Convolution Neural Network method was carried out for the detection and the classification of objects. Comparative experiments were conducted on various benchmark datasets and methods that showed improved accurate detection and classification results.Keywords
Cite This Article
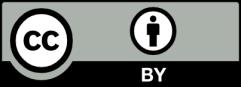