Open Access
ARTICLE
Intelligent Smart Grid Stability Predictive Model for Cyber-Physical Energy Systems
1 Department of Computer Science and Information Systems, College of Applied Sciences, AlMaarefa University, Ad Diriyah, Riyadh, 13713, Kingdom of Saudi Arabia
2 Department of Computer Engineering, College of Computers and Information Technology, Taif University, Taif, 21944, Kingdom of Saudi Arabia
3 Department of Archives and Communication, King Faisal University, Al Ahsa, Hofuf, 31982, Kingdom of Saudi Arabia
* Corresponding Author: Ashit Kumar Dutta. Email:
Computer Systems Science and Engineering 2023, 44(2), 1219-1231. https://doi.org/10.32604/csse.2023.026467
Received 27 December 2021; Accepted 15 February 2022; Issue published 15 June 2022
Abstract
A cyber physical energy system (CPES) involves a combination of processing, network, and physical processes. The smart grid plays a vital role in the CPES model where information technology (IT) can be related to the physical system. At the same time, the machine learning (ML) models find useful for the smart grids integrated into the CPES for effective decision making. Also, the smart grids using ML and deep learning (DL) models are anticipated to lessen the requirement of placing many power plants for electricity utilization. In this aspect, this study designs optimal multi-head attention based bidirectional long short term memory (OMHA-MBLSTM) technique for smart grid stability prediction in CPES. The proposed OMHA-MBLSTM technique involves three subprocesses such as pre-processing, prediction, and hyperparameter optimization. The OMHA-MBLSTM technique employs min-max normalization as a pre-processing step. Besides, the MBLSTM model is applied for the prediction of stability level of the smart grids in CPES. At the same time, the moth swarm algorithm (MHA) is utilized for optimally modifying the hyperparameters involved in the MBLSTM model. To ensure the enhanced outcomes of the OMHA-MBLSTM technique, a series of simulations were carried out and the results are inspected under several aspects. The experimental results pointed out the better outcomes of the OMHA-MBLSTM technique over the recent models.Keywords
Cite This Article
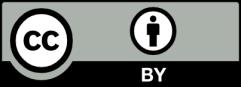