Open Access
ARTICLE
Deep Learning with Optimal Hierarchical Spiking Neural Network for Medical Image Classification
1 Department of Computer Science and Engineering, Anjalai Ammal Mahalingam Engineering College, Koilvenni, 614403, Tamil Nadu, India
2 Department of CSE, E.G.S. Pillay Engineering College, Nagapattinam, 611002, Tamil Nadu, India
* Corresponding Author: P. Immaculate Rexi Jenifer. Email:
Computer Systems Science and Engineering 2023, 44(2), 1081-1097. https://doi.org/10.32604/csse.2023.026128
Received 16 December 2021; Accepted 24 January 2022; Issue published 15 June 2022
Abstract
Medical image classification becomes a vital part of the design of computer aided diagnosis (CAD) models. The conventional CAD models are majorly dependent upon the shapes, colors, and/or textures that are problem oriented and exhibited complementary in medical images. The recently developed deep learning (DL) approaches pave an efficient method of constructing dedicated models for classification problems. But the maximum resolution of medical images and small datasets, DL models are facing the issues of increased computation cost. In this aspect, this paper presents a deep convolutional neural network with hierarchical spiking neural network (DCNN-HSNN) for medical image classification. The proposed DCNN-HSNN technique aims to detect and classify the existence of diseases using medical images. In addition, region growing segmentation technique is involved to determine the infected regions in the medical image. Moreover, NADAM optimizer with DCNN based Capsule Network (CapsNet) approach is used for feature extraction and derived a collection of feature vectors. Furthermore, the shark smell optimization algorithm (SSA) based HSNN approach is utilized for classification process. In order to validate the better performance of the DCNN-HSNN technique, a wide range of simulations take place against HIS2828 and ISIC2017 datasets. The experimental results highlighted the effectiveness of the DCNN-HSNN technique over the recent techniques interms of different measures. Please type your abstract here.Keywords
Cite This Article
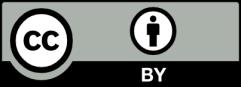