Open Access
ARTICLE
Rice Bacterial Infection Detection Using Ensemble Technique on Unmanned Aerial Vehicles Images
Department of Computer and Information Science, Faculty of Applied Science, King Mongkut’s University of Technology North Bangkok, 1518 Pracharat 1 Rd., Wongsawang, Bangsue, Bangkok, 10800, Thailand
* Corresponding Author: Sathit Prasomphan. Email:
Computer Systems Science and Engineering 2023, 44(2), 991-1007. https://doi.org/10.32604/csse.2023.025452
Received 24 November 2021; Accepted 19 January 2022; Issue published 15 June 2022
Abstract
Establishing a system for measuring plant health and bacterial infection is critical in agriculture. Previously, the farmers themselves, who observed them with their eyes and relied on their experience in analysis, which could have been incorrect. Plant inspection can determine which plants reflect the quantity of green light and near-infrared using infrared light, both visible and eye using a drone. The goal of this study was to create algorithms for assessing bacterial infections in rice using images from unmanned aerial vehicles (UAVs) with an ensemble classification technique. Convolution neural networks in unmanned aerial vehicles image were used. To convey this interest, the rice’s health and bacterial infection inside the photo were detected. The project entailed using pictures to identify bacterial illnesses in rice. The shape and distinct characteristics of each infection were observed. Rice symptoms were defined using machine learning and image processing techniques. Two steps of a convolution neural network based on an image from a UAV were used in this study to determine whether this area will be affected by bacteria. The proposed algorithms can be utilized to classify the types of rice deceases with an accuracy rate of 89.84 percent.Keywords
Cite This Article
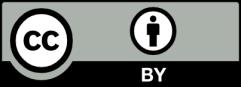
This work is licensed under a Creative Commons Attribution 4.0 International License , which permits unrestricted use, distribution, and reproduction in any medium, provided the original work is properly cited.