Open Access
ARTICLE
Novel Contiguous Cross Propagation Neural Network Built CAD for Lung Cancer
1 Department of Electronics & Communication Engineering, Government College of Engineering, Tirunelveli, 627007, Tamilnadu, India
2 Department of Electrical & Electronics Engineering, University College of Engineering, Ramanathapuram, 623513, Tamilnadu, India
* Corresponding Author: A. Alice Blessie. Email:
Computer Systems Science and Engineering 2023, 44(2), 1467-1484. https://doi.org/10.32604/csse.2023.025399
Received 22 November 2021; Accepted 16 February 2022; Issue published 15 June 2022
Abstract
The present progress of visual-based detection of the diseased area of a malady plays an essential part in the medical field. In that case, the image processing is performed to improve the image data, wherein it inhibits unintended distortion of image features or it enhances further processing in various applications and fields. This helps to show better results especially for diagnosing diseases. Of late the early prediction of cancer is necessary to prevent disease-causing problems. This work is proposed to identify lung cancer using lung computed tomography (CT) scan images. It helps to identify cancer cells’ affected areas. In the present work, the original input image from Lung Image Database Consortium (LIDC) typically suffers from noise problems. To overcome this, the Gabor filter used for image processing is highly enhanced. In the next stage, the Spherical Iterative Refinement Clustering (SIRC) algorithm identifies cancer-suspected areas on the CT scan image. This approach can help radiologists and medical experts recognize cancer diseases and syndromes so that serious progress can be avoided in the early stages. These new methods help to remove unwanted portions of the CT image and better utilization the image. The subspace extraction of features approach is beneficial for evaluating lung cancer. This paper introduces a novel approach called Contiguous Cross Propagation Neural Network that tends to locate regions afflicted by lung cancer using CT scan pictures (CCPNN). By using the feature values from the fourth step of the procedure, the proposed CCPNN tends to categorize the lesion in the lung nodular site. The efficiency of the suggested CCPNN approach is evaluated using classification metrics such as recall (%), precision (%), F-measure (percent), and accuracy (%). Finally, the incorrect classification ratios are determined to compare the trained networks’ effectiveness, through these parameters of CCPNN, it obtains the outstanding performance of 98.06% and it has provided the lowest false ratio of 1.8%.Keywords
Cite This Article
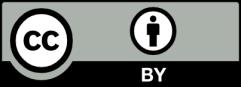
This work is licensed under a Creative Commons Attribution 4.0 International License , which permits unrestricted use, distribution, and reproduction in any medium, provided the original work is properly cited.