Open Access
ARTICLE
Dynamic Ensemble Multivariate Time Series Forecasting Model for PM2.5
School of Computing, SASTRA University, Thanjavur, 613401, India
* Corresponding Author: Umamakeswari Arumugam. Email:
Computer Systems Science and Engineering 2023, 44(2), 979-989. https://doi.org/10.32604/csse.2023.024943
Received 05 November 2021; Accepted 27 December 2021; Issue published 15 June 2022
Abstract
In forecasting real time environmental factors, large data is needed to analyse the pattern behind the data values. Air pollution is a major threat towards developing countries and it is proliferating every year. Many methods in time series prediction and deep learning models to estimate the severity of air pollution. Each independent variable contributing towards pollution is necessary to analyse the trend behind the air pollution in that particular locality. This approach selects multivariate time series and coalesce a real time updatable autoregressive model to forecast Particulate matter (PM) PM2.5. To perform experimental analysis the data from the Central Pollution Control Board (CPCB) is used. Prediction is carried out for Chennai with seven locations and estimated PM’s using the weighted ensemble method. Proposed method for air pollution prediction unveiled effective and moored performance in long term prediction. Dynamic budge with high weighted k-models are used simultaneously and devising an ensemble helps to achieve stable forecasting. Computational time of ensemble decreases with parallel processing in each sub model. Weighted ensemble model shows high performance in long term prediction when compared to the traditional time series models like Vector Auto-Regression (VAR), Autoregressive Integrated with Moving Average (ARIMA), Autoregressive Moving Average with Extended terms (ARMEX). Evaluation metrics like Root Mean Square Error (RMSE), Mean Absolute Error (MAE) and the time to achieve the time series are compared.Keywords
Cite This Article
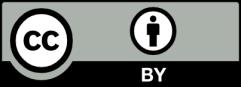
This work is licensed under a Creative Commons Attribution 4.0 International License , which permits unrestricted use, distribution, and reproduction in any medium, provided the original work is properly cited.